Comparing Theories for the Maintenance of Late LTP and Long-Term Memory: Computational Analysis of the Roles of Kinase Feedback Pathways and Synaptic Reactivation
- 1Department of Neurobiology and Anatomy, W.M. Keck Center for the Neurobiology of Learning and Memory, McGovern Medical School at the University of Texas Health Science Center at Houston, Houston, TX, United States
- 2Engineering and Medicine, Texas A&M Health Science Center, Houston, TX, United States
A fundamental neuroscience question is how memories are maintained from days to a lifetime, given turnover of proteins that underlie expression of long-term synaptic potentiation (LTP) or “tag” synapses as eligible for LTP. A likely solution relies on synaptic positive feedback loops, prominently including persistent activation of Ca2+/calmodulin kinase II (CaMKII) and self-activated synthesis of protein kinase M ζ (PKMζ). Data also suggest positive feedback based on recurrent synaptic reactivation within neuron assemblies, or engrams, is necessary to maintain memories. The relative importance of these mechanisms is controversial. To explore the likelihood that each mechanism is necessary or sufficient to maintain memory, we simulated maintenance of LTP with a simplified model incorporating persistent kinase activation, synaptic tagging, and preferential reactivation of strong synapses, and analyzed implications of recent data. We simulated three model variants, each maintaining LTP with one feedback loop: autonomous, self-activated PKMζ synthesis (model variant I); self-activated CamKII (model variant II); and recurrent reactivation of strengthened synapses (model variant III). Variant I predicts that, for successful maintenance of LTP, either 1) PKMζ contributes to synaptic tagging, or 2) a low constitutive tag level persists during maintenance independent of PKMζ, or 3) maintenance of LTP is independent of tagging. Variant II maintains LTP and suggests persistent CaMKII activation could maintain PKMζ activity, a feedforward interaction not previously considered. However, we note data challenging the CaMKII feedback loop. In Variant III synaptic reactivation drives, and thus predicts, recurrent or persistent activation of CamKII and other necessary kinases, plausibly contributing to persistent elevation of PKMζ levels. Reactivation is thus predicted to sustain recurrent rounds of synaptic tagging and incorporation of plasticity-related proteins. We also suggest (model variant IV) that synaptic reactivation and autonomous kinase activation could synergistically maintain LTP. We propose experiments that could discriminate these maintenance mechanisms.
Introduction
Long-term memory (LTM) lasting days or more necessitates synaptic structural changes and strengthening, and de novo protein synthesis (Davis and Squire, 1984; Kandel, 2001; Mayford et al., 2012). Long-term potentiation (LTP), and the invertebrate analog long-term synaptic facilitation (LTF), are likely necessary for many types of memory formation (Lynch, 2004; Nabavi et al., 2014; Mirisis et al., 2016; Asok et al., 2019). Late LTP (L-LTP, lasting > ~2 h) requires protein synthesis (Stanton and Sarvey, 1984; Frey et al., 1998; Abraham and Williams, 2008) and can last at least a year (Abraham et al., 2002). Thus a fundamental concern is to determine mechanisms that preserve LTM for months or even a lifespan (Vanyushin et al., 1974; Crick, 1984; Schwartz, 1993; Holliday, 1999; Roberson and Sweatt, 1999).
One hypothesized mechanism involves persistent upregulation of local translation, or enhanced incorporation, of synaptic proteins termed plasticity-related proteins (PRPs; Figure 1) (Fonkeu et al., 2019). Increased translation or incorporation would be based on positive feedback loops specific to synapses strengthened to participate in LTM storage (Smolen et al., 2019). As illustrated in models, a common element of such positive feedback is a bistable switch in which the strength or weight of specific synapses, and biophysical variables such as kinase activities, switch post-stimulus from basal to persistently increased values (Lisman, 1985; Bhalla and Iyengar, 1999; Song et al., 2007; Zhang et al., 2010, 2016). Although synapse-specific mechanisms for maintenance of LTM may be supplemented by cell-wide mechanisms, such as upregulation of specific genes (e.g., Kyrke-Smith and Williams, 2018) or neuronal excitability (Mozzachiodi and Byrne, 2010), here we explore the necessity, sufficiency, and interactions among proposed synapse-specific positive feedback loops to maintain LTP. Synapse-specific feedback loops are necessary. In their absence, given protein turnover time scales of hours to days (Eden et al., 2011; Cohen et al., 2013), homeostatic processes (Turrigiano et al., 1998; Abbott and Nelson, 2000) and relaxation would average out synaptic strengths, erasing LTM, LTP, or LTF. We focus on proposed mechanisms for maintenance of NMDA-dependent, excitatory LTP. Mechanisms for long-term plasticity at other synapse types may differ (Freeman, 2015; Hawkins and Byrne, 2015; Hige, 2018; Lamsa and Lau, 2019).
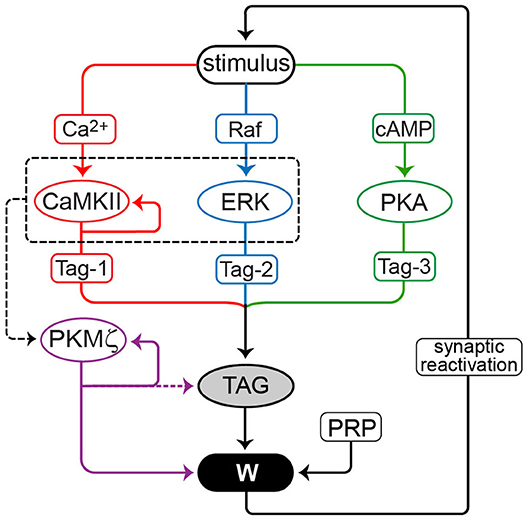
Figure 1. Model for maintenance of LTP. Three model variants contrast positive feedback loops mediated by CaMKII, PKMζ and synaptic reactivation. Stimuli activate CaMKII, PKA, Raf, and ERK. CaMKII, ERK, and PKA phosphorylate a synaptic tag. CaMKII, and ERK also converge (dashed box and arrow) to induce translation of PKMζ. Production of plasticity-related protein (PRP) is necessary to maintain LTP. TAG, PRP, and PKMζ converge to increase synaptic weight W. The feedback loops are: (1) PKMζ can enhance its own translation and thus activity. For this model variant, two cases are simulated: (a) PKMζ contributes to increasing W, but not to maintaining TAG, and (b) PKMζ also contributes to maintaining an elevated level of TAG (dashed arrow). (2) Active CaMKII can reinforce and maintain its own activity. (3) Ongoing synaptic reactivation. An increase in W enhances the amplitude of spontaneous LTP-reinforcing stimuli. Only for potentiated W is the stimulus amplitude sufficient to significantly reactivate downstream signaling pathways.
Essential kinases for LTP induction include protein kinase A (PKA) (Abel et al., 1997; Matsushita et al., 2001), the atypical PKC denoted protein kinase M ζ (PKMζ) (Sacktor et al., 1993), the MAP kinase (MAPK) isoform ERK (Rosenblum et al., 2002), and CaM kinase II (CaMKII) (Malenka et al., 1989; Otmakhov et al., 1997). Smolen et al. (2019) discuss hypothesized positive feedback involving ERK and other kinases. Here we limit our focus to CaMKII and PKMζ.
CaMKII
This feedback loop relies on self-sustaining autophosphorylation of CaMKII holoenzymes resulting in autonomous activation (Lisman and Goldring, 1988), which has generated, in models, a bistable switch of CaMKII activity and synaptic strength (Zhabotinsky, 2000; Miller et al., 2005; Aslam et al., 2009). Active CaMKII can enhance translation (Atkins et al., 2004, 2005). Persistence of CaMKII activation, and the necessity of CaMKII activation for maintaining L-LTP and LTM, has been challenged (Lengyel et al., 2004; see Smolen et al., 2019 for review). A light-inducible inhibitor of CaMKII was used by Murakoshi et al. (2017) who argued persistent CaMKII activity is not necessary to maintain LTP. However, this study did not specifically examine whether CaMKII inhibition blocks L-LTP at late times (>2 h post-stimulus) or late LTM (~24 h post-training). Thus, it is still possible that autonomously active CaMKII, perhaps in a small postsynaptic zone, maintains L-LTP and LTM. Saneyoshi et al. (2019) delineated a positive feedback loop that could contribute to localized, persistent CaMKII activation. CaMKII phosphorylates Tiam1, a Rac guanine nucleotide exchange factor. Binding of Tiam1 to CaMKII is thereby enhanced, and Tiam1, in turn, occludes the autoinhibitory domain of CaMKII in a manner that enhances autonomous activity of CaMKII. A caveat is that the activity of CaMKII was not monitored for more than 40 min post-stimulus, preventing direct application of this study to L-LTP maintenance. A study using dominant negative (dn) CaMKII also suggests persistent CaMKII activity is required to maintain spatial LTM (Rossetti et al., 2017), although with a caveat that the molecular mechanism by which dnCaMKII disrupts LTM is not known and may not depend on activity per se. Considering all these data, it remains plausible that persistently enhanced CamKII activity is important to maintain some forms of L-LTP and LTM, although further study is needed.
PKMζ
This positive feedback loop relies on a self-sustaining increase in translation, and thus activity, of PKMζ. Inhibiting PKMζ blocks maintenance of L-LTP and several forms of LTM (Serrano et al., 2005, 2008; Pastalkova et al., 2006; Shema et al., 2007). Following spatial memory formation, PKMζ activity is increased for at least a month (Hsieh et al., 2017). PKMζ upregulates its own synthesis (Kelly et al., 2007), forming a positive feedback loop. Modeling has suggested this loop can generate a bistable switch (Smolen et al., 2012; Jalil et al., 2015; Helfer and Shultz, 2018). As output, PKMζ promotes AMPAR trafficking to synapses (Migues et al., 2010), which could maintain increased synaptic strength. PKMζ also phosphorylates the histone acetyltransferase denoted CREB binding protein, which may enhance transcription required for late maintenance of LTM (Ko et al., 2016). However, the requirement for persistently enhanced PKMζ activity has been questioned by observations of normal LTP and LTM following constitutive (Lee et al., 2013) or conditional (Volk et al., 2013) PKMζ knockouts. But another study (Wang et al., 2016) found, in contrast, impaired maintenance of LTM and LTP following PKMζ knockdown. Thus, it is plausible that persistently enhanced PKMζ activity is necessary for the maintenance of some forms of L-LTP and LTM. But if PKMζ activity is persistent, is it due to autonomous positive feedback, or is it maintained due to another process such as synaptic reactivation or persistent CamKII activity?
Reactivation
Specific assemblies of neurons, or memory engrams, are activated upon learning and during memory retrieval (Zhou et al., 2009; Park et al., 2016) and specific patterns of functional connectivity among neurons within an engram, based on potentiated synapses as well as neuronal excitability, are important for memory storage (Mozzachiodi and Byrne, 2010; Liu et al., 2012; Ryan et al., 2015; Josselyn et al., 2017; Choi et al., 2018; Tonegawa et al., 2018; Josselyn and Tonegawa, 2020). Recurrent reactivation of neurons within engrams encoding recent experience, commonly termed replay, appears generic (Ikegaya et al., 2004; Miller et al., 2014; Wu and Foster, 2014) and correlates with improved LTM (Schapiro et al., 2018).
Such reactivation of engram synapses may be necessary to maintain late LTM. Inducible forebrain-restricted knockdown of the NR1 subunit of the NMDA receptor, several months post-training, disrupts retention of remote cued and contextual fear memories and of taste memory (see also Shimizu et al., 2000; Cui et al., 2004, 2005). An implication of NR1 necessity is that recurrent reactivation of engram neurons and synapses connecting them induces Ca2+ influx through NMDA receptors and voltage-dependent Ca2+ channels, thereby reactivating processes, such as kinase activation, that originally induced LTP. By these processes, reactivation could generate recurring “rounds” of LTP, reinforcing and preserving L-LTP and LTM (Fauth and van Rossum, 2019). Recently, disrupting reactivation during sleep of hippocampal neurons associated with a specific environment was found to impair memory for goal location within that environment (Gridchyn et al., 2020).
In this scenario kinases would be persistently or recurrently active, and make essential contributions in maintaining LTM. However, in contrast to the feedback loops discussed previously, persistent activation of CaMKII, PKMζ, or other kinases would not be self-sustaining. Synaptic reactivation, and consequent kinase activation, would also likely induce transcription via stimulus-responsive factors such as cAMP response element binding protein (CREB) (Smolen et al., 2019). Resultant global increases in synaptic proteins may be a required permissive factor for reinforcement of L-LTP.
Summing up the above, evidence suggests self-sustaining kinase activation, in particular persistently elevated activities of CaMKII or PKMζ, may be necessary for the maintenance of LTM. There is also evidence that synaptic reactivation may be necessary. But a further question is, are any of these positive feedback mechanisms also sufficient for maintenance? A problem in addressing sufficiency is that these feedback loops are interlocked such that manipulation of one process affects others. One way to address this issue is to develop a mathematical model of the processes and modify the parameters of each process, and thereby examine conditions under which each process is necessary and sufficient for LTM.
We developed four variants of a simplified model, to compare qualitative dynamics of kinase activities and synaptic strength predicted by these positive feedback loops. Two interrelated but contrasting hypotheses were continued: (1) autonomous activation of one or more kinases such as PKMζ or CaMKII is necessary and sufficient to maintain L-LTP and LTM, and (2) persistent kinase activation is necessary, but it depends on synaptic reactivation, which is sufficient. Simulations of these mechanisms suggest experiments that can examine dynamics predicted by these hypotheses. In two model variants, bistable switches of kinase activation are generated, respectively by (1) persistently increased PKMζ synthesis and activity, or (2) persistent CaMKII activation. In a third variant bistability in synaptic weight is generated by positive feedback in which synaptic reactivation preferentially occurs at strengthened synapses. L-LTP maintenance requires recurrent kinase activation downstream of reactivation. A fourth variant illustrates that synaptic reactivation and autonomous kinase activation could synergistically maintain L-LTP.
Model Development
The model describes dynamics of kinases necessary for L-LTP induction, and represents kinase-dependent setting of a synaptic “tag” that has been shown to be necessary for localized “capture” of plasticity-related proteins necessary for L-LTP (Frey and Morris, 1997, 1998). This model is simplified from those we have previously published (Smolen et al., 2006, 2014) in that it focuses on synapse-specific positive feedback loops. It does not represent gene induction and transcriptional or epigenetic positive feedback, although these have been hypothesized as permissive factors for maintaining memory (Zhang et al., 2016; Smolen et al., 2019).
Figure 1 schematizes the model. Stimuli, such as a tetanus, are represented by concurrent brief elevations of synaptic Ca2+ and cAMP, and brief activation of Raf kinase upstream of ERK. Stimulus parameters, including durations, are provided in Methods and are similar to Smolen et al. (2014). Some intermediate processes, such as interaction of Ca2+ with calmodulin or calmodulin with CaMKII, and Ras activation upstream of Raf, are not included. CaMKII, PKA, and ERK activate a synaptic tag to place a synapse in a labile state capable of “capturing” plasticity factors (proteins or mRNAs) to increase synaptic strength. Parameters describing kinetics of enzyme activation are given in Methods. Data support the importance of these kinases for tag setting. At least one tag modification appears to be mediated by PKA (Barco et al., 2002). Postsynaptic CAMKII activity is required for L-LTP, and tag setting is blocked by inhibition of CaMKII (Redondo et al., 2010). CaMKII stimulates translation via phosphorylation of cytoplasmic polyadenylation element binding protein, a candidate for Tag-1 (Atkins et al., 2004, 2005). ERK is also necessary for LTP (English and Sweatt, 1997; Rosenblum et al., 2002). In the model, tagging requires phosphorylation of three sites; Tag-1, Tag-2, and Tag-3; respectively, substrates of CAMKII, PKA, and synaptic ERK. We assume multiplicative dependence of synaptic tagging on these phosphorylations, thus the tag (variable TAG) is the product of amounts of phosphorylated Tag-1–Tag-3. The molecular nature of the synaptic tag is not characterized. However, assumed multiplicative dependence is consistent with block of tag setting by inhibition of a single kinase, CaMKII (Redondo et al., 2010), and with the requirement of ERK activity for LTP (Rosenblum et al., 2002).
CaMKII and ERK are assumed to cooperate to induce PKMζ synthesis, because inhibition of these kinases impairs PKMζ translation (Kelly et al., 2007). PKMζ translation is increased by LTP induction (Hernandez et al., 2003), and PKMζ concentrates at potentiated synapses (Hsieh et al., 2017). Stimulus-induced increases in TAG and PKMζ are assumed to converge, with these variables multiplying to determine the rate of increase of synaptic weight, denoted W. Increased W corresponds to L-LTP. Without positive feedback, W decays to baseline over several hours. We include as a parameter the level of a generic plasticity-related protein (PRP) required for LTP. A multiplicative dependence for the rate of increase of W is assumed, with this rate proportional to PRP, TAG, and PKMζ. This multiplicative dependence is an assumption and a phenomenological representation of the net effect of numerous processes. It is chosen to represent the separate necessity of each of the components (TAG, PRP, and PKMζ) which must act in concert to support L-LTP induction. There is also a small basal rate of increase in W, to yield positive basal W in the absence of stimulus.
Three forms of positive feedback are represented in distinct model variants, I–III, respectively. Each type of feedback is able, on its own, to generate robust bistability in W, maintaining L-LTP. Simulations consider one type of feedback at a time. These feedback loops (Figure 1) are: (1) Self-sustaining synthesis of PKMζ. Persistent activity is implemented as a Hill function in which active PKMζ feeds back to increase the rate of its own synthesis, with activity necessary and sufficient for maintaining increased W. This feedback could serve as a proxy for more detailed mechanisms, such as repression by PKMζ of the translation inhibitor Pin1 (Westmark et al., 2010). (2) Self-sustaining CaMKII autoactivation. Persistent activity is implemented as a Hill function in which active CaMKII feeds back to increase the rate of its own activation. This feedback could serve as a proxy for mechanisms including incorporation and activation of new subunits in a CaMKII holoenzyme (Miller et al., 2005) or reciprocal activation of CaMKII and Tiam1 (Saneyoshi et al., 2019). (3) Spontaneous reactivation in which a potentiated synapse is subject to brief, repeated elevations of Ca2+ and cAMP. Long-lasting kinase activation requires synaptic reactivation. Reactivation amplitudes are assumed to be greater at stronger synapses (i.e., the amplitudes of these brief Ca2+ and cAMP elevations increase with synaptic weight W). Therefore, reactivation is only effective at increasing W, counteracting its spontaneous decay, at synapses that are already strong. Reactivation stimuli, which only need to generate minor increases in W to counteract slow passive decay, are assumed weaker than the stimulus that induces L-LTP. Equations and parameters are given in Methods.
To maintain L-LTP in the model, TAG must remain elevated to some extent, either periodically (due to synaptic reactivation) or constitutively. TAG elevation allows a slow ongoing incorporation of plasticity-related protein, increasing synaptic weight to counter passive weight decay. The elevation of TAG required during LTP maintenance remains well below the peak value of TAG during LTP induction.
Results
Simulated L-LTP Maintenance Due to Self-Sustaining Kinase Activation
L-LTP Induction
We first turned off all positive feedback loops, simulating only induction (Figures 2A1,A2). We simulated a standard induction protocol—three 1-s tetani with interstimulus intervals of 5 min. CaMKII responds rapidly to each Ca2+ increase and deactivates in ~1 min, similar to the time course in dendritic spines (Lee et al., 2009), whereas synaptic ERK activity, and PKA activity, accumulate over tetani. The synaptic tag variable TAG increases by a large relative factor from basal to peak, due to the multiplicative convergence of ERK, PKA, and CaMKII activities to increase TAG. In alternate simulations in which an additive kinase interaction was assumed, the relative increase in TAG was much lower, resulting in excessive basal levels and in a lack of effective block of LTP upon single kinase inhibition (not shown). The synaptic weight W increases for > 1 h, consistent with data that L-LTP takes >1 h to develop when induced by chemical stimuli that bypass early LTP (Ying et al., 2002). The magnitude of L-LTP was assessed 2 h after the last tetanus, as the increase in W relative to pre-stimulus. Standard values for all parameters yielded L-LTP of 131%. Empirical excitatory postsynaptic potential increases after three or four tetani have similar values (English and Sweatt, 1997, Woo et al., 2000). Without positive feedback, L-LTP decayed with W returning to near basal ~10 h post-stimulus. This time scale for decay of W is set by the time constant τltp (Methods, Equation 21), and was chosen to allow comparison of model dynamics when L-LTP was maintained by the feedback mechanisms proposed above.
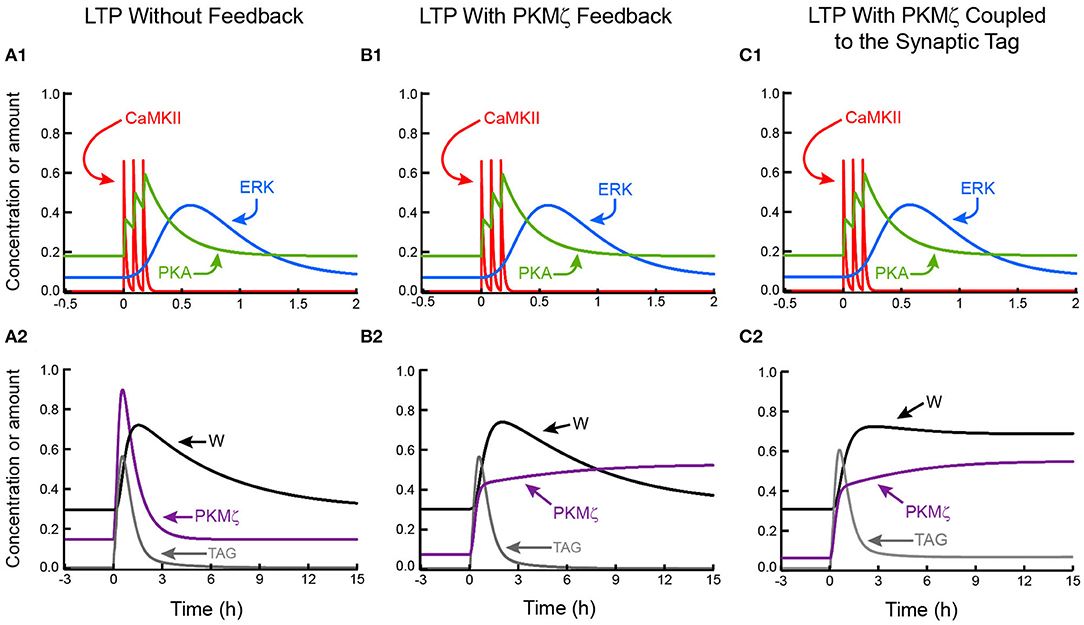
Figure 2. Simulated LTP, without and with positive feedback based on sustained PKMζ activity. (A1) A three-tetanus stimulus induces peaks of CaMKII activity and elevates activities of PKA and ERK. Without positive feedback, kinase activities return to basal with the slowest variable, ERK, elevated for ~1.5 h. (A2) Increased kinase activities activate TAG and PKMζ. LTP decays over h. (B1) With persistent PKMζ activation, a tetanic stimulus leads to only temporary activation of CaMKII, ERK, and PKA. (B2) Enhancement by PKMζ of its own synthesis and activity leads to bistability and a state switch for PKMζ. But TAG is not persistently elevated and LTP is not maintained. (C1,C2) If PKMζ is assumed to increase the level of TAG, then stimulus-induced persistent PKMζ activation leads to elevation of TAG and maintenance of LTP. In Figures 2–5, time courses of some variables are vertically scaled for ease of visualization. Scaling factors are given in Methods.
Self-Sustained Elevation of PKMζ Synthesis
In Figures 2B1,B2, this positive feedback loop sustains a bistable switch, with PKMζ persistently active. All other dynamic variables, including TAG and W, decay to basal levels post-stimulus. L-LTP is not maintained. This lack of maintenance follows from the lack of dependence of TAG on PKMζ phosphorylation. Without elevated TAG, PRP cannot be incorporated. Thus, an important implication, and topic for future empirical investigation, is that if self-sustained activity of PKMζ is sufficient to maintain LTP, then either: (1) the tag is not needed for maintenance, or (2) PKMζ activation participates in and is sufficient for persistent synaptic tagging, or (3) there is a constitutive basal level of the synaptic tag, independent of kinase activation and sufficient to maintain L-LTP. In cases (2) and (3), as noted above, the low level of TAG required for maintenance may not conflict with empirical observations of synaptic tag decay. Activation of metabotropic glutamate receptors could allow PKMζ to participate in synaptic tagging (Li et al., 2014).
To illustrate maintenance of L-LTP dependent on activation of the synaptic tag by PKMζ, we repeated the simulation of Figure 2B with the equation giving the level of TAG (Equation 17, Methods) modified. A positive term was added to the right-hand side, kTPKMPKM. This is the simplest plausible dependence. It assumes the level of TAG and the activity of PKMζ equilibrate rapidly compared to changes in PKMζ. As illustrated in Figures 2C1,C2, this PKMζ dependence suffices for persistent maintenance of L-LTP.
During maintenance, minor but persistent elevation of TAG above its pre-stimulus baseline is evident. This elevation is minor because the other kinases that contribute to tag setting (CaMKII, ERK, PKA) are not persistently activated. Minor TAG elevation suffices to allow continued slow incorporation of plasticity-related protein to counter passive synaptic weight decay. This TAG elevation is much less than the peak of TAG during L-LTP induction. It may not be readily evident empirically, and thus may not conflict with data suggesting a tag lifetime of 2–3 h (Frey and Morris, 1997, 1998). In this simulation, for bistability in W and TAG to be evident, it is essential to assume that PKMζ activity increases TAG additively, with the kTPKMPKM term independent of CaMKII, ERK, and PKA. If, instead, PKMζ activity simply multiplies together with the activities of CaMKII, ERK, and PKA, then the persistent elevation of TAG and W is negligible, because of the return of CaMKII activity to its very low basal value.
The model can also simulate maintenance of L-LTP for the case in which a low, basal level of synaptic tagging is assumed to persist, independent of the activity of all modeled kinases (not shown). In this case, the maintained increase in synaptic weight W is entirely due to the persistent increase in PKMζ activity.
Self-Sustained Autoactivation of CaMKII
To simulate maintenance of L-LTP due to self-sustained activation of CaMKII, PKMζ autoactivation was removed and feedback mechanism two was implemented (Methods, Equation 23). In Figures 3A1,A2, this feedback sustains a bistable switch, with CaMKII activity and W persistently active. ERK and PKA activities still decay. Similarly to Figure 2C2, TAG remains persistently elevated. This elevation is again well below the peak during induction. Intriguingly, PKMζ activity also remains elevated. CaMKII increases synthesis and activity of PKMζ (Kelly et al., 2007). Thus, in this model variant, PKMζ activity depends on CaMKII-catalyzed phosphorylation (Equations 18 and 20, Methods), so that both activities are concurrently enhanced. Persistent PKMζ activity is not autonomously self-sustaining. However, as noted in the Introduction, the hypothesis that autonomous CaMKII activity sustains L-LTP has substantial empirical challenges.
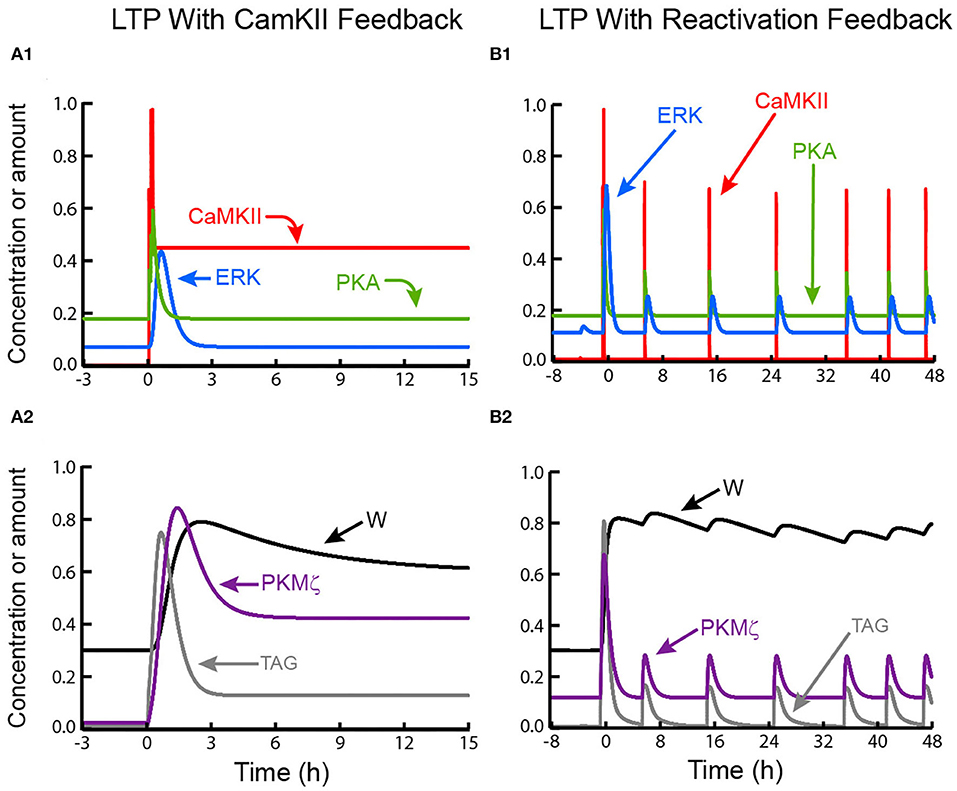
Figure 3. Simulated LTP with positive feedback based on sustained CaMKII activity or on synaptic reactivation. (A1,A2) LTP maintenance due to autoactivation of CaMKII. (B1,B2) Maintenance due to positive feedback in which a strengthened synapse undergoes reactivation events, with each event reinforcing LTP. In both these model variants, PKMζ does not act to increase the level of TAG.
Simulated Synaptic Reactivation Maintains L-LTP by Reactivating KINASES and Tagging
Maintaining L-LTP by reactivation of a strengthened synapse (feedback mechanism 3) yields qualitatively different dynamics. As illustrated in Figures 3B1,3B2, recurrent increases of cAMP, Ca2+, and Raf activity are induced by reactivations (Methods, Equations 25–27). These increases activate ERK, PKA, and CaMKII, synthesize PKMζ, and elevate TAG. These increases were adjusted by trial and error to maintain a stable, although fluctuating, state of increased synaptic strength. During reactivation, peak elevations of kinase activities excepting CaMKII, and of the synaptic tag, are substantially less than during LTP induction, and TAG relaxes to low levels between reactivations. If, as assumed here, reactivations are not very frequent, then these dynamics may be compatible with empirically reported decay of the tag within ~1–2 h after LTP induction. These simulations illustrate a model property more general than this specific reactivation loop. Sustained or recurrent activation of all simulated kinases is necessary and sufficient to maintain a degree of synaptic tagging and maintain L-LTP.
The simulation of Figures 3B1,B2 thus indicates a way in which LTP could be maintained in the absence of autonomous biochemical feedback loops. A qualitative difference between this maintenance mechanism and those based on autonomous kinase activation is that here, the synaptic weight can exhibit significant oscillations depending on the frequency of reactivation, whereas weights may be more stable with automous kinase activation.
The Model Is Not Unduly Sensitive to Most Parameter Variations
The sensitivity of the stimulus response—the amount of L-LTP—to relatively minor changes (±15%) of all parameter values including stimulus amplitudes was analyzed, perturbing from the standard values (Methods). There are 43 parameters, yielding 86 parameter variations for each model variant (note for each variant, 2–3 parameters governing strength of positive feedback are set to zero, thus these parameter variations are zero). We used a standard analysis defining a set of relative sensitivities Si, with i ranging over all parameters pi (Frank, 1978). R denotes the magnitude of stimulus response. For each pi, a change is made, and the resulting change in R is determined. Relative sensitivity Si is defined as the absolute value of the relative, or fractional, change in R divided by the relative change in pi,
R is the magnitude of L-LTP after tetani. The sensitivity of four simulations was assessed—without feedback (Figure 2A), with kinase feedback (Figures 2B, 3A), and with reactivation feedback (Figure 3B). For Figures 2A,B, which were not bistable in W, L-LTP was quantified 2 h post-stimulus, whereas for the bistable simulations of Figures 3A,B, L-LTP was quantified 50 h post-stimulus.
Sensitivity to most of the 86 parameter variations was not large, with Si below 3. There were 13 exceptions with Si between 3.0 and 9.9. Interestingly, 10 of these exceptions were for Raf-ERK pathway parameters (Methods, Equations 6–13), and these 10 were >3 for all four simulations. These exceptions were: 15% increases and decreases in the total concentration of Raf kinase ([Raftot], Equation 6 in Methods), in the rate constant for Raf activation (kfbasRaf, first paragraph of Methods and Equation 7), in the rate constants for MEK activation (kfMEK) and inactivation (kbMEK), and in the inactivation rate constant for Raf (kbRaf). Two other exceptions were for ERK rate constants (+kfERK, -kbERK). Finally, the simulation with PKMζ autoactivation (Figure 2B) had Si = 7.0 for a decrease in the corresponding Hill constant (Kpkm, Equation 24). This lack of large sensitivity for most parameter variations (73 out of 86) suggests model variants I—III are relatively robust and can be regarded as reasonable qualitative models of synaptic feedback mechanisms. Further studies might seek to add buffering mechanisms, especially within the Raf-ERK pathway, to reduce sensitivity to the specific parameters noted.
Simulations Suggest a Test to Distinguish Feedback Mechanisms for Maintenance of L-LTP
Further simulations suggest the consequences of inhibition of key kinases, in particular PKMζ or CaMKII, may provide an empirical test to distinguish between feedback mechanisms. Figure 4A illustrates that if self-sustained activation of CaMKII is sufficient to maintain L-LTP, then transient inhibition of a different kinase, in this case PKMζ, would not abolish L-LTP maintenance. W repotentiates after inhibition is removed, because CaMKII autoactivation does not require PKMζ activity and persists throughout inhibition. In contrast to this simulation, empirical inhibition of PKMζ does appear to inhibit L-LTP and LTM (Ling et al., 2002; Shema et al., 2007; Serrano et al., 2008), suggesting self-sustained CaMKII activation is not sufficient. However CaMKII autoactivation could still be necessary as some data suggests (Sanhueza et al., 2007; Rossetti et al., 2017) and as we simulated (model variant II).
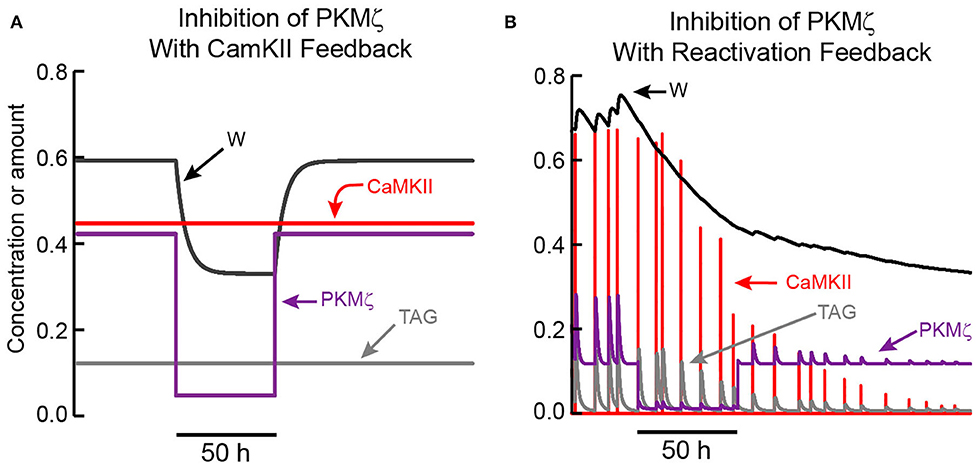
Figure 4. Simulated effects of PKMζ inhibition on LTP maintenance. (A) When LTP is maintained by CaMKII activation, only a temporary reduction in synaptic weight occurs. TAG does not depend on PKMζ and remains elevated. Thus, following PKMζ inhibition, TAG and PKMζ can again cooperate to increase W, restoring LTP. (B) With positive feedback based on reactivation of strong synapses, a decrease in W, induced by PKMζ inhibition, decreases amplitudes of reactivation stimuli and activation of CaMKII and other kinases, decreasing TAG. Long-lasting inhibition of PKMζ (90% inhibition for 50 h) decreases W below a threshold, such that the bistable system transitions to the basal state with low W, PKMζ, and TAG. In this model variant, one slow time constant is assumed to describe synaptic weight decay. Because of this slow time constant, the inhibition duration required to decrease W below threshold is ~ 2 d.
Figure 4B illustrates that if recurrent synaptic reactivation maintains L-LTP, then inhibition of PKMζ can permanently reverse L-LTP. W declines while PKMζ is inhibited, because PKMζ activity is needed to maintain elevated synaptic weight. Therefore, if W declines sufficiently during PKMζ inhibition, selective reactivation of a strong synapse is no longer present, and LTP cannot be maintained. Even if inhibition of PKMζ is then removed, W continues to decline toward its basal value (Figure 4B). For standard parameter values (Methods), PKMζ inhibition does need to last ~ 50 h in order for W to decrease sufficiently, so that after inhibition removal reactivation is not able to re-potentiate W. Empirically, PKMζ inhibition is likely to reduce synaptic weight more rapidly than would a simple loss of reactivation, in which case a shorter inhibition duration would suffice. This simulation argues that empirically, inhibition of PKMζ during the maintenance phase of L-LTP does not enable discrimination between: (A) the sufficiency of a feedback loop based on autonomous, activity-independent PKMζ activation, vs. (B) the necessity, or even sufficiency, of a reactivation feedback loop involving PKMζ as an essential component.
Synaptic Reactivation and Autonomous Kinase Activation Could Synergistically Maintain L-LTP
The preceding model variants contrast qualitative dynamics that characterize maintenance of L-LTP and LTM due to one of two plausible forms of positive feedback, autonomous kinase activation and synaptic reactivation. It is also possible that multiple positive feedback loops act in concert to sustain bistability in synaptic weight, maintaining L-LTP and LTM. To explore dynamics associated with this scenario, we constructed a fourth model variant (Methods). We modified model variant III, based on synaptic reactivation, to include self-sustaining enhancement of PKMζ translation as in variant I. Parameters determining the strength of both forms of feedback were reduced from their standard values, so that neither reactivation nor PKMζ activation alone could maintain L-LTP. However, when both forms of positive feedback operated concurrently, bistability resulted in PKMζ activity and in W, and L-LTP was maintained.
Figures 5A1,A2 illustrates induction of L-LTP and its maintenance post-tetanus. These dynamics are similar to Figure 3B. During L-LTP maintenance, activities of CaMKII, ERK, and PKA, along with the synaptic tag, fluctuate above basal levels. At 2,000 min post-stimulus PKMζ positive feedback was switched off. W, and PKMζ activity, both decline to basal levels (with a slower time constant for W), illustrating that reactivation alone cannot sustain bistability. Figure 5B illustrates an analogous simulation, for which the PKMζ positive feedback remains on and reactivation is switched off. Bistability is again lost. PKMζ activity and W decline to basal levels, although the decline is quite slow for the simulated parameter values.
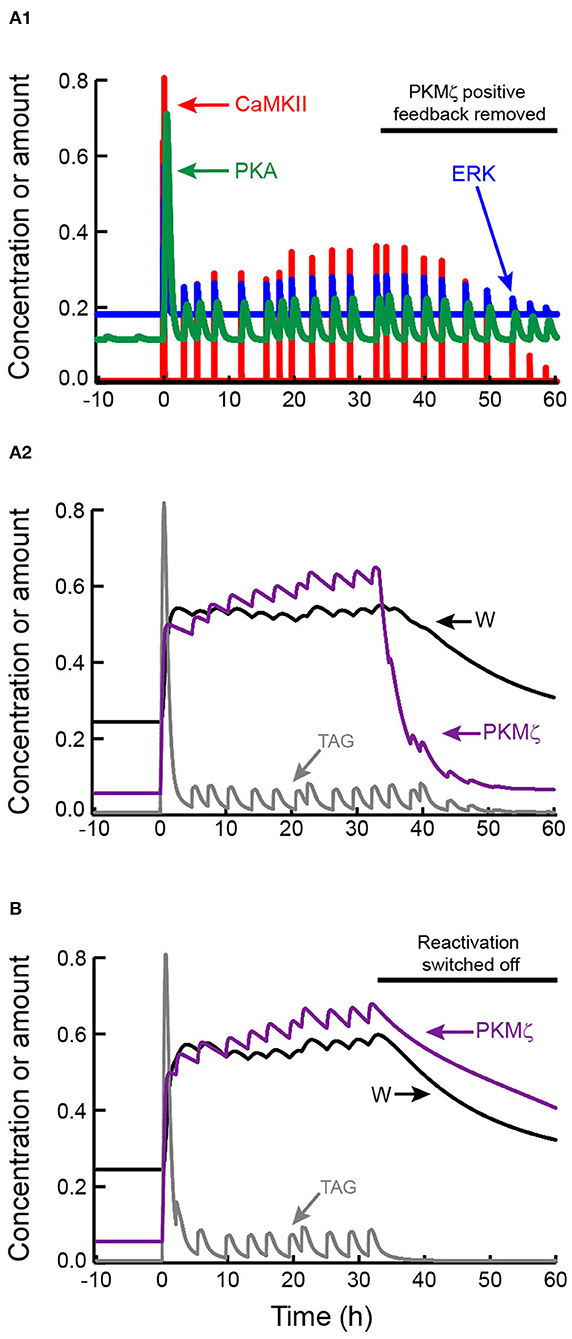
Figure 5. Maintenance of L-LTP by mutual reinforcement of self-sustaining PKMζ activity and synaptic reactivation. (A1,A2) Tetanic stimulus induces kinase activation, synaptic tagging, and persistent increase in W. After W increases, reactivation leads to recurrent increases in CaMKII, ERK, and PKA activities in (A1), and TAG in (A2), but these variables remain well below peak values during LTP induction. Starting at t = 33 h (black horizontal bar), PKMζ positive feedback is switched off by setting the parameter kPKM to zero. Despite continued synaptic reactivation, PKMζ and W decline to basal levels. (B) LTP is induced and maintained with the same parameters as in (A), with only the random timing of reactivation events differing. Starting at t = 33 h (black horizontal bar), reactivation is switched off, while PKMζ positive feedback remains on. PKMζ and W decline slowly to basal levels.
Discussion
We have considered two interrelated hypotheses: (1) autonomous self-sustaining activation of a kinase, focusing on PKMζ or CaMKII, is necessary and sufficient to maintain L-LTP; and (2) persistent activation of kinases is not autonomous, and is recurrently induced by synaptic reactivation, which maintains L-LTP. There are empirical data in favor of each hypothesis. Modeling investigated whether the dynamics of kinase activities, synaptic tagging, and synaptic weight predicted by these hypotheses could be discriminated. We identified dynamic differences, and make empirical predictions that could further discriminate these mechanisms.
Dynamics of Autonomous Kinase Activity
L-LTP was maintained by positive feedback based on autonomous CaMKII activation (Figure 2C), or based on synaptic reactivation (Figure 3A), or based on autonomously increased synthesis of active PKMζ (Figure 2C). With autonomous CaMKII activation, CaMKII activity remains high during maintenance, well above the other kinases. In contrast, when L-LTP is maintained by persistent PKMζ activation or by synaptic reactivation, CaMKII activity is maintained at a lower level, sufficient to sustain, in cooperation with other kinases, a low persistent level of TAG that allows continued necessary incorporation of plasticity-related proteins (PRPs). In these latter two cases, low CaMKII activity suffices because its cooperating kinase, PKMζ, is persistently or recurrently elevated.
Model variant I, based on autonomous, self-sustaining synthesis of active PKMζ did not initially maintain L-LTP (Figure 2B), because we first assumed there was no persistence of the synaptic tag considered essential for incorporation of plasticity related proteins. However, when PKMζ activity was assumed to elevate TAG, L-LTP was maintained (Figure 2C). With this model variant, L-LTP could also be maintained if a low basal level of TAG, independent of the kinase activities in the model, was assumed to persist, or if L-LTP maintenance was assumed to be independent of tagging.
If a positive feedback loop based on intrinsic activation of a kinase, such as CaMKII or PKMζ, is necessary and sufficient for maintenance of L-LTP, then the simulation of Figure 4A illustrates that inhibition of a kinase or process not within the feedback loop should fail to permanently reverse L-LTP. If sustained activity of PKMζ is both necessary and sufficient, then inhibiting CaMKII should have no effect, and also vice versa. Assuming inhibitor specificity, the empirical loss of established L-LTP and LTM upon PKMζ inhibition implies that no feedback loop (intrinsic or network based) independent of PKMζ can be both necessary and sufficient for maintenance.
We note additional positive feedback loops have been proposed to maintain LTM (Smolen et al., 2019). For example, in mammals and in Aplysia, isoforms of the translation activator denoted cytoplasmic polyadenylation element binding protein (CPEB) can upregulate translation at synapses that have undergone LTP or LTF. Aggregation of CPEB and increased translation has been hypothesized to be self-perpetuating and to maintain synaptic strength (Si et al., 2003, 2010; Drisaldi et al., 2015; Fioriti et al., 2015; Rayman and Kandel, 2017; Li et al., 2018). However, data suggesting that ongoing synaptic reactivation is necessary in mammals (Shimizu et al., 2000; Cui et al., 2004, 2005) imply that autonomous CPEB aggregation would not be sufficient.
We have not further explored the possible consequences of a low basal TAG independent of kinase activities. However, it is qualitatively evident that in this case, any persistent activation of a self-sustaining kinase or other process that increases PRP levels could maintain L-LTP, via enhanced PRP incorporation driven by basal TAG. For example, if CPEB aggregation increased translation of PRPs, then a basal TAG level could suffice to facilitate incorporation of these PRPs, maintaining L-LTP. Persistent CaMKII activity could upregulate PRP translation via CPEB phosphorylation (Atkins et al., 2004, 2005), maintaining L-LTP.
Dynamics of Synaptic Reactivation
In model variants III-IV for which recurrent synaptic reactivation is essential, only potentiated synapses undergo substantial reactivation to reinforce L-LTP. This assumption needs to be empirically verified. If synaptic reactivation is stronger or more frequent at potentiated synapses in memory engrams, then reactivation is predicted to yield increased time-average activity of kinases, specific to potentiated synapses, and reinforcing LTP. As with LTP induction, these kinases include ERK, CaMKII, PKA, and plausibly PKMζ. Monitoring FRET-engineered substrates of these kinases over relatively long periods (h or more) might demonstrate increased activity. In addition, simulated dynamics of synaptic reactivation (Figure 3B) differ from those of autonomous kinase activation (Figures 2C, 3A) in that reactivation events generate substantial fluctuations in activities of synaptic CaMKII, ERK, and the level of TAG (Figure 3B). Such fluctuations may be observable empirically. Our model has not addressed whether the frequency of reactivation events would be increased at potentiated synapses. To model reactivation frequency would require a neuronal network model within which a synaptic memory engram can be stored and maintained, and reactivation events can be self-consistently generated.
It would be highly desirable to strengthen the empirical case for the necessity of synaptic reactivation by examining the consequences, for maintenance of L-LTP and LTM, of additional pharmacological or genetic methods for prolonged synaptic activity reduction. Because reported timescales on which reactivation appears necessary are long—weeks to months—such studies will need to be done in vivo rather than with in vitro preparations of limited duration. In vitro, over hours, synaptic activity patterns may not recapitulate patterns in vivo, reducing the likelihood of positive feedback loop based on reactivation of specific synapses. Biochemical positive feedback, such as persistent kinase activation, may contribute to maintain L-LTP over hours in vitro.
Although the present study has focused on engram-level reactivation, another form of reactivation could be mediated by autocrine actions. For example, brain-derived neurotrophic factor (BDNF) released by synaptic activity can act, via TrkB receptors, to reactivate biochemical pathways necessary for LTP, including the Ras → Raf → MEK → ERK signaling cascade (Panja and Bramham, 2014). These events can upregulate transcription of bdnf, generating a positive feedback loop (Bambah-Mukku et al., 2014). We note that recurrent engram-level reactivation would be expected to upregulate BDNF secretion (Kolarow et al., 2007), with secreted BDNF reinforcing LTP (Kovalchuk et al., 2002; Barco et al., 2005). A modeling study does suggest positive feedback in which BDNF upregulates bdnf expression may play a role in maintaining L-LTP (Zhang et al., 2016).
Predictions to Help Discriminate Between Proposed Maintenance Mechanisms
Figure 3A2 illustrates sustained activation can depend on a separate process in a way that complicates determination of which process is necessary. Here, persistent activation of PKMζ occurs, but it is not autonomous. Persistent PKMζ activity depends on self-sustaining activation of CaMKII, which enhances PKMζ synthesis. These dynamics suggest that if CaMKII activation maintains L-LTP, this maintenance could be due in part to downstream PKMζ activation. If so, a prediction is that if CaMKII is inhibited, PKMζ levels at potentiated synapses would decay. In contrast, if PKMζ activity is elevated due to autonomous biochemical feedback, elevated synthesis and activity of synaptic PKMζ may not be reversed by inhibition of CaMKII, or by inhibition of synaptic reactivation.
Simulations of the model variant for which selective reactivation of strong synapses maintains L-LTP (Figure 4B) predict that for this mechanism, inhibition of any kinase will permanently reverse L-LTP only if inhibition lasts long enough to decrease synaptic weight such that synapse-specific reactivation no longer occurs, and LTP is not reinforced. In this simulation PKMζ was inhibited, but the same argument applies for inhibition of CaMKII or any other kinase necessary for L-LTP. Prolonged, neuron-specific inhibition of kinases such as ERK, PKA, or CaMKII may provide a test of this prediction.
When we modeled L-LTP maintenance as dependent on synaptic reactivation, elevation of PKMζ activity was still assumed necessary for maintenance, but this elevation was due to synaptic reactivation and was not autonomous. For this case, without autonomy, empirical inhibition of synaptic activity would be predicted to knock down PKMζ levels.
It appears difficult to empirically separate a model in which PKMζ positive feedback and synaptic reactivation are both necessary to sustain L-LTP (Figure 5) from reliance on reactivation alone (Figure 3B). The difficulty occurs because for both variants, inhibiting either PKMζ or synaptic reactivation abolishes bistability and maintenance of L-LTP.
Our model is limited in what it can infer concerning the dependence of L-LTP maintenance on protein turnover rates. For CaMKII, our assumed fast deactivation time constant (1 min) is consistent with observed rapid kinetics of CaMKII deactivation (Murakoshi et al., 2017), but far slower than protein turnover. Half-lives of synaptic CaMKII α, δ, and γ isoforms have been measured to be 3–4 days (Cohen et al., 2013), and a more complex model would be needed to simulate CaMKII turnover. Such a model would need to represent CaMKII subunit turnover and replacement within a CaMKII holoenzyme, and also account for the likelihood that a persistently active population of postsynaptic CaMKII should be modeled as a separate pool. Singh and Bhalla (2018) have recently modeled turnover and subunit exchange among CaMKII holoenzymes and simulated bistability, with persistence of enhanced CaMKII activity for at least 60 days. As regards maintenance of L-LTP by recurrent synaptic reactivation (model variant III), this maintenance mechanism is relatively insensitive to protein turnover rates. Kinases, and the synaptic tag, can deactivate or decay relatively quickly, as long as reactivation events occur frequently enough so that reactivation of kinases, and possibly increased translation of postsynaptic proteins, induces periodic elevations of TAG and synaptic weight to counteract ongoing decay.
As regards maintenance of L-LTP by bistable PKMζ activity (model variant I) our model assumes a standard value of 0.02 min−1 for the PKMζ deactivation rate constant. If deactivation corresponds to degradation, then the degradation time constant is 50 min. Cohen et al. (2013) did not measure the turnover time of synaptic PKMζ, and as far as we know it has not yet been determined. Eden et al. (2011) measured half-lives of ~100 proteins from a human cancer cell line and found values ranging from 45 min to 23 h. These data suggest that if PKMζ deactivation corresponds to degradation, our assumed rate constant is at the upper end of plausible values. With the current model, we are not able to substantially decrease this rate constant without impairing model dynamics. If the PKMζ degradation time is assumed to be substantially longer, e.g., several days as found for the majority of synaptic proteins (Cohen et al., 2013), a more complex model would be needed. Such a model might assume a relatively rapid sequestration of constitutively active PKMζ, or an inactivating modification of PKMζ followed by slow degradation.
A basic assumption of our illustrated simulations is that processes necessary for the induction of L-LTP are also necessary for maintenance of L-LTP of engram synapses. This assumption will need to be empirically tested. We assumed the synaptic tag necessary for “capture” of plasticity-related proteins (PRPs) during induction of L-LTP remains essential during maintenance of L-LTP to allow reinforcing PRP capture. Indeed, Shema et al. (2011) previously suggested that persistent, selective upregulation of tagging at strong synapses may capture and localize PKMζ at those synapses, helping to maintain synaptic strength. If this assumption is correct, the tag is predicted to be persistently set to some extent, or periodically reactivated, at strong synapses. During maintenance, the activities of CaMKII, ERK, and PKMζ are all predicted to be necessary insofar as these kinases participate in tag setting, or enhance synaptic AMPAR incorporation. But autonomous bistability in these activities may (model variants I, II, IV) or may not (variant III) be necessary.
The tag identity has yet to be established, but several molecular species have been suggested to participate including the BDNF receptor TrkB (Lu et al., 2011) and polymerized actin (Ramachandran and Frey, 2009). One or more of these species is predicted to be persistently, or recurrently, upregulated at strong synapses. But the extent of upregulation during L-LTP maintenance, required to counter passive synaptic weight decay, may be substantially less than the increase during induction of L-LTP (Figures 2C2, 3A2,B2). Thus, it may be difficult to empirically demonstrate tag persistence or recurrence. Nonetheless, a promising strategy to further delineate the feedback mechanism(s) responsible for maintaining L-LTP and LTM is to investigate whether, and which, kinase activities or suggested tagging molecules are upregulated selectively at strengthened engram synapses.
Methods
Equations Prior to Positive Feedback
In the model, LTP is induced by three tetanic stimuli with a standard inter-stimulus interval (ISI) of 5 min. As in our previous study (Smolen et al., 2006), each individual tetanus is modeled as inducing: (1) A square-wave increase in synaptic Ca2+ concentration, [Casyn], to a peak value ACasyn. The brief increase has a duration Cadur, with a standard value of 3 s that is similar to data (Malenka et al., 1992, Pologruto et al., 2004). Otherwise, Ca2+ remains at a basal value Cabas; (2) An increase in cAMP concentration [cAMP] to a value AcAMP, with duration dcAMP. dcAMP has a standard value of 1 min, similar to data (Bacskai et al., 1993; Vincent and Brusciano, 2001). Otherwise cAMP is at cAMPbas; (3) An increase in the zeroth-order rate constant for activation of Raf kinase by its upstream effector Ras. This rate constant is denoted kfRaf and has a basal value of kfbasRaf. Upon stimulus it increases to (ASTIM + kfbasRaf). The duration of increase does not appear constrained by data. We assumed a plausible duration of 1 min.
CaMKII is assumed to be activated by a fourth-power Hill function of cytoplasmic Ca2+ concentration. This Hill functions constitutes a minimal representation of the activation of CaM kinases by calmodulin (CaM), because four Ca2+ ions bind cooperatively to CaM and CaM-Ca4 activates CaM kinases. Data show a steep [Ca2+] dependence that can be characterized by a Hill coefficient ≥4 (Bradshaw et al., 2003).
The following ordinary differential equation (ODE) results for activation of CaMKII.
For cAMP to activate PKA, two cAMP molecules must bind cooperatively to the regulatory subunit of a PKA holoenzyme (Herberg et al., 1996). Thus, our qualitative representation of PKA activation assumes the activation rate is a Hill function of the second power of [cAMP].
In Equation 4, the time constant τPKA is given a value of 15 min, resulting in substantial accumulation of PKA activity over three tetani with interstimulus intervals of 5 min.
At the synapse, Raf kinase is phosphorylated and activated by stimuli, and by a small basal rate constant.
MAP kinase kinase, or MEK, is phosphorylated and activated by Raf. In turn, MEK activates ERK. Saturable Michaelis-Menten terms have been commonly used to describe phosphorylations and dephosphorylations of the kinases in the ERK cascade (e.g., Pettigrew et al., 2005), and are used here. Phosphatase dynamics are not explicitly modeled. Only doubly phosphorylated MEK and ERK are considered active. Concentrations of singly phosphorylated forms are given by conservation conditions.
As discussed previously (Smolen et al., 2006, 2014), data suggest that CaMKII, PKA, and ERK all play roles in setting the synaptic tag required for induction of late LTP. The molecular nature of the tag has not been characterized. We assume three phosphorylation sites, one for each kinase, and represent the amount of active synaptic tag as the product of the fractional phosphorylations of each site. The differential equations for the dynamics of these fractional phosphorylations, and their multiplication to give the level of active tag, are as in Smolen et al. (2014).
PKMζ is translated after stimulus and is constitutively active, with activity denoted PKMact. Its translation is increased by activation of CaMKII and ERK. As a minimal representation of these dynamics, CaMKII and ERK are each assumed to phosphorylate sites denoted PCK2 and PERK. The rate of synthesis of active PKMζ is assumed proportional to the product of PCK2 and PERK, along with a small basal rate of synthesis, ktransbaspkm.
As in Smolen et al. (2006), the model hypothesizes a limiting factor, a protein Plim, to prevent the synaptic weight W from increasing indefinitely with repeated simulation. As W increases, Plim is posited to decrease via incorporation into the synapse. The rate of increase of W is given as a product of the amount of TAG, the concentration of the plasticity-related protein PRP, the activity of PKMζ, and the concentration of the limiting protein Plim. There is also a small basal rate of increase to balance ongoing decay.
The rate of Plim consumption is assumed to increase as the rate of W increases, yielding a similar ODE. However, because PKMζ is assumed to increase W by enhancing AMPAR incorporation into the synapse (Migues et al., 2010), PKMζ is not included in the ODE for Plim (i.e., Plim is not taken to be AMPAR).
Simulation of Positive Feedback
Equations 2–22 as written describe the induction of late LTP (L-LTP) that decays over several hours, without any positive feedback to sustain L-LTP indefinitely. In simulations of persistent L-LTP, three types of feedback were implemented: (1) Sustained autoactivation of CaMKII, (2) Sustained autoactivation of PKMζ, or (3) Ongoing reactivation of a potentiated synapse, resulting in recurrent reinforcement of L-LTP. Only one feedback type was implemented at a time. These feedback types were, respectively, implemented as follows, by modifying the above equations:
Type 1: The right-hand side of Equation 3, describing the rate of activation of CaMKII, was increased by adding a quadratic Hill function describing autoactivation,
Type 2: The right-hand side of Equation 20, describing the rate of activation of PKMζ, was increased by adding a quadratic Hill function describing autoactivation,
Type 3: Equations 2–22 were unaltered. Instead, ongoing brief, spontaneous elevations of [Casyn], [cAMP], and the rate constant kfRaf for Raf activation were added. Similarly to our previous study (Smolen, 2007), positive feedback was implemented by making the amplitudes of these elevations proportional to an increasing Hill function of synaptic weight. For this qualitative model, the Hill function was chosen to be fairly steep (power of 5) so that only potentiated synapses undergo significant reactivation. During each brief reactivation event, the elevated levels of [Casyn], [cAMP], and kfRaf are,
The elevation duration was 3 s for Casyn and 1 min for cAMP and kfRaf. Outside of elevations [Casyn] = Cabas, [cAMP] = cAMPbas, and kfRaf = kfbasRaf.
In the absence of detailed data, the frequency of these reactivations was set low enough so that the synaptic tag variable TAG would relax to near its basal value between reactivations, to accord with current data that the tag decays within ~90 min after LTP induction (Frey and Morris, 1997, 1998). The frequency was also set high enough to allow W to be maintained in an upper state for a reasonable value of the synaptic weight decay time constant τltp. To implement this, we used a Gaussian random variable for the intervals between successive reactivations. This variable was updated for each new reactivation, using the Box-Mueller algorithm. The mean interval was 500 min and the standard deviation was 150 min. A minimum interval of 10 min was imposed.
To simulate only induction, not maintenance, of L-LTP (Figures 2A1,A2), Equations 23–27 were omitted.
Parameter Values and Numerical Methods
Standard parameter values are used in all simulations except as noted otherwise. Without positive feedback, these are as follows:
Cabas = 0.04 μM, cAMPbas = 0.06 μM, Cadur = 3 s, dcAMP = 1 min, ACasyn = 0.8 μM, AcAMP = 0.25 μM, ASTIM = 0.13 min−1.
Kcasyn = 0.7 μM, kfck2 = 180 μM min−1, τck2 = 1 min, Kcamp = 1.0 μM, τPKA = 15 min.
RAFTOT = MEKTOT = ERKTOT = 0.25 μM, kfbasRaf = 0.0075 min−1, kbRaf = 0.12 min−1, kfMEK = 0.6 min−1, kbMEK = 0.025 μM min−1, KMEK = 0.25 μM, kfERK = 0.52 min−1, kbERK = 0.025 μM min−1, KERK = 0.25 μM.
ktranspkm = 0.2 μM min−1, ktransbaspkm = 0.0015 μM min−1, kdpkm = 0.02 min−1.
kphos1 = 0.15 μM−1 min−1, kdeph1 = 0.008 min−1, kphos2 = 0.8 μM−1 min−1, kdeph2 = 0.2 min−1, kphos3 = 0.06 μM−1 min−1, kdeph3 = 0.05 min−1, kphos4 = 0.1 μM−1 min−1, kdeph4 = 0.1 min−1, kphos5 = 2.0 μM−1 min−1, kdeph5 = 0.1 min−1.
[PRP] = 1.0 μM, kltp = 500 μM−2 min−1, kltpbas = 0.01 min−1, τltp = 300 min, Klim = 0.2 μM, kPl = 6.0 min−1, kPlbas = 0.0035 μM min−1, τPl = 100 min.
Positive feedback introduces new parameters (Equations 23–27). For positive feedback via CaMKII autoactivation, or via PKMζ autoactivation, new standard parameter values are, kCaMKII = 4.0 μM min−1, KCaMKII = 1.0 μM, kPKM = 0.028 μM min−1, KPKM = 0.75 μM. Also, kltp = 70 μM−2 min−1 for CaMKII autoactivation, and 300 μM−2 min−1 for the simulation of Figure 2B with PKMζ autoactivation. For the simulation of Figure 2C with PKMζ increasing the synaptic tag, kltp = 240 μM−2 min−1, and the coupling coefficient kTPKM = 0.0001 μM−1.
For feedback via synaptic reactivation kltp = 480 μM−2 min−1, KW = 4.0. τltp was increased to 3200 min and kltpbas was correspondingly decreased, to 0.00086 min−1.
Model variant IV (Figure 5) used the parameter values of the synaptic reactivation simulation (Figure 3C) with the following exceptions. Reactivation events were weakened by reducing AcAMP, ACasyn, and ASTIM (Equations 25–27) to 80% of their standard values. PKMζ positive feedback was included (Equation 24), but the coupling constant kPKM was reduced to 0.0078 μM min−1. These changes ensured neither form of positive feedback could sustain bistability on its own. Other changes were: ktransbaspkm = 0.00045 μM min−1, kdpkm = 0.006 min−1, kltp = 180 μM−2 min−1, kltpbas = 0.003 min−1, τltp = 800 min. The mean and standard deviation of reactivation frequency were (200 min, 50 min).
For some parameters, empirical data were used to determine standard values. As described in Smolen et al. (2006), the model's total concentrations of Raf, MEK, and ERK, and the peak concentration of active PKA as determined by stimulus and kinetic parameters, are similar to experimental values. The peak concentration of active CaMKII due to a tetanus is approximately 10% of the estimated total CaMKII concentration (Bhalla and Iyengar, 1999). Data were also used to constrain the amplitude and duration of Ca2+ and cAMP elevations (Smolen et al., 2006).
However, for many parameters, such as biochemical rate constants in vivo, data are lacking to determine values. Therefore, these parameters were chosen by trial and error to yield dynamics similar to empirical time courses, when the latter are available. In simulations that do not assume bistable CaMKII activity, CaMKII decays with a time constant of ~1 min. This brief lifetime is consistent with data describing CaMKII activity in dendritic spines (Murakoshi et al., 2017). PKA activity increases by ~150% during L-LTP induction, and decays post-tetanus with a time constant of 15 min. This amplitude, and decay time constant, are consistent with data (Roberson and Sweatt, 1996). Simulated postsynaptic ERK activation lasts ~90 min post-tetanus. This duration is plausible but is bracketed by empirical data. One study suggests ERK remains phosphorylated for as long as 8 h after tetanus (Ahmed and Frey, 2005). However, other studies (English and Sweatt, 1997; Liu et al., 1999) suggest briefer activation of ~30 min. Because long-lasting ERK activity could regulate transcription important for L-LTP, we suggest further study of ERK kinetics is warranted. After tetanus the synaptic tag (variable TAG) returns to baseline, or decays to a persistent but low activity to maintain L-LTP, within ~3 h. This decay time is similar to data (Frey and Morris, 1997, 1998). L-LTP induction (the increase of W) is complete in ~2 h, similar to the time required for empirical induction of L-LTP with BDNF (bypassing early LTP) (Ying et al., 2002). Parameters in the phenomological representations of positive feedback loops (CaMKII autoactivation, persistent PKMζ activity, and synaptic reactivation) in Equations 23–27 are not determined by empirical data. Rather, values for these parameters were chosen by trial and error to yield bistable switches of synaptic weight that were induced by simulated tetani, but with both stable states robust to perturbations (weak stimuli). The resulting standard parameter value set cannot be claimed to be unique, and the simulated time courses of some variables, such as the individual TAG substrates Tag-1–Tag-3, are not constrained by data.
In Figures 2–5, some variables are vertically scaled, scale factors are as follows. Figures 2A1,A2: 0.12 for [CaMKIIact], 7.0 for [ERKPP], 50.0 for [PKAact], 0.1 for W, 700.0 for TAG, 2.0 for [PKMact]. Figures 2B1,B2, same as Figures 2A1,A2 except 0.6 for [PKMact]. Figures 2C1,C2, same as Figures 2B1,B2. Figures 3A1,3A2, same as Figures 2A1,A2 except 0.3 for [PKMact]. Figures 3B1,B2: 0.12 for [CaMKIIact], 11.0 for [ERKPP], 50.0 for [PKAact], 0.1 for W, 1000.0 for TAG, 1.5 for [PKMact]. Figure 4A, same as Figures 3A1,3A2. Figure 4B, same as Figures 3B1,3B2. Figure 5, same as Figure 3B except 0.75 for [PKMact].
The forward Euler method was used to integrate ODEs and, for the simulations of Figures 2A,B, we verified that using the fourth-order Runge-Kutta method did not yield significant differences. The time step was 10 ms. Model variables were initialized to 0.0001 (μM or arbitrary units) excepting variables given by conservation conditions. Prior to stimulus, model variables were equilibrated for at least two simulated days. The slowest variables, W and [PKMact], were set to equilibrium basal values determined by other variables, except for Figures 4A,B for which W and [PKMact], were initialized in their upper state. Models were programmed in Java.
Java programs to reproduce the simulations in Figures 2A,B, 3A,B have been deposited in the Github online database (https://github.com/psmolen0/Smolen_Byrne_LTP_Model), as files fig2ab3a.java and fig3b.java. These programs use model variants I-III, and will also be deposited in ModelDB. These programs are also included as supplementary manuscript files.
Data Availability Statement
The original contributions presented in the study are included in the article/supplementary materials, further inquiries can be directed to the corresponding author/s.
Author Contributions
PS and JB conceived and directed the project. PS developed the model and carried out simulations. PS, DB, and JB participated in analyzing results and writing the manuscript. All authors contributed to the article and approved the submitted version.
Funding
This study was supported by National Institutes of Health grant NS102490. Other than general grant support, the funder had no role in directing the research or preparing the manuscript.
Conflict of Interest
The authors declare that the research was conducted in the absence of any commercial or financial relationships that could be construed as a potential conflict of interest.
Acknowledgments
We thank H. Shouval and W. Sossin for comments on an earlier version of the manuscript.
References
Abbott, L. F., and Nelson, S. B. (2000). Synaptic plasticity: taming the beast. Nat. Neurosci. 3, 1178–1183. doi: 10.1038/81453
Abel, T., Nguyen, P. V., Barad, M., Deuel, T. A., Kandel, E. R., and Bourtchouladze, R. (1997). Genetic demonstration of a role for PKA in the late phase of LTP and in hippocampus-based long-term memory. Cell 88, 615–626. doi: 10.1016/S0092-8674(00)81904-2
Abraham, W. C., Logan, B., Greenwood, J. M., and Dragunow, M. (2002). Induction and experience-dependent consolidation of stable long-term potentiation lasting months in the hippocampus. J. Neurosci. 22, 9626–9634. doi: 10.1523/JNEUROSCI.22-21-09626.2002
Abraham, W. C., and Williams, J. M. (2008). LTP maintenance and its protein synthesis-dependence. Neurobiol. Learn Mem. 89, 260–268. doi: 10.1016/j.nlm.2007.10.001
Ahmed, T., and Frey, J. U. (2005). Plasticity-specific phosphorylation of CAMKII, MAP-kinases, and CREB during late-LTP in rat hippocampal slices in vitro. Neuropharmacology 49, 477–492. doi: 10.1016/j.neuropharm.2005.04.018
Aslam, N., Kubota, Y., Wells, D., and Shouval, H. Z. (2009). Translational switch for long-term maintenance of synaptic plasticity. Mol. Syst. Biol. 5:284. doi: 10.1038/msb.2009.38
Asok, A., Leroy, F., Rayman, J. B., and Kandel, E. R. (2019). Molecular mechanisms of the memory trace. Trends Neurosci. 42, 14–22. doi: 10.1016/j.tins.2018.10.005
Atkins, C. M., Davare, M. A., Oh, M. C., Derkach, V., and Soderling, T. R. (2005). Bidirectional regulation of cytoplasmic polyadenylation element-binding protein phosphorylation by Ca2+/calmodulin – dependent protein kinase II and protein phosphatase I during hippocampal long-term potentiation. J. Neurosci. 25, 5604–5610. doi: 10.1523/JNEUROSCI.5051-04.2005
Atkins, C. M., Nozaki, N., Shigeri, Y., and Soderling, T. R. (2004). Cytoplasmic polyadenylation element binding protein-dependent protein synthesis is regulated by calcium/calmodulin-dependent protein kinase II. J. Neurosci. 24, 5193–5201. doi: 10.1523/JNEUROSCI.0854-04.2004
Bacskai, B. J., Hochner, B., Mahuat-Smith, M., Adams, S. R., Kaang, B., Kandel, E. R., et al. (1993). Spatially resolved dynamics of cAMP and protein kinase A subunits in Aplysia sensory neurons. Science 260, 222–226. doi: 10.1126/science.7682336
Bambah-Mukku, D., Travaglia, A., Chen, D. Y., Pollonini, G., and Alberini, C. M. (2014). A positive autoregulatory BDNF feedback loop via C/EBPβ mediates hippocampal memory consolidation. J. Neurosci. 34, 12547–12559. doi: 10.1523/JNEUROSCI.0324-14.2014
Barco, A., Alarcon, J. M., and Kandel, E. R. (2002). Expression of constitutively active CREB facilitates the late phase of long-term potentiation by enhancing synaptic capture. Cell 108, 689–703. doi: 10.1016/S0092-8674(02)00657-8
Barco, A., Patterson, S. L., Alarcon, J. M., Gromova, P., Mata-Roig, M., Morozov, A., et al. (2005). Gene expression profiling of facilitated L-LTP in VP16-CREB mice reveals that BDNF is critical for the maintenance of LTP and its synaptic capture. Neuron 48, 123–137. doi: 10.1016/j.neuron.2005.09.005
Bhalla, U. S., and Iyengar, R. (1999). Emergent properties of networks of biological signaling pathways. Science 283, 381–387. doi: 10.1126/science.283.5400.381
Bradshaw, J. M., Kubota, Y., Meyer, T., and Schulmann, H. (2003). An ultrasensitive Ca2+/calmodulin-dependent protein kinase II-protein phosphatase 1 switch facilitates specificity in postsynaptic calcium signaling. Proc. Natl. Acad. Sci. U.S.A. 100, 10512–10517. doi: 10.1073/pnas.1932759100
Choi, J. H., Sim, S. E., Kim, J. I., Choi, D. I., Oh, J., Ye, S., et al. (2018). Interregional synaptic maps among engram cells underlie memory formation. Science 360, 430–435. doi: 10.1126/science.aas9204
Cohen, L. D., Zuchman, R., Sorokina, O., Muller, A., Dieterich, D. C., Armstrong, J. D., et al. (2013). Metabolic turnover of synaptic proteins: kinetics, interdependencies and implications for synaptic maintenance. PLoS ONE 8:e63191. doi: 10.1371/journal.pone.0063191
Cui, Z., Lindl, K. A., Mei, B., Zhang, S., and Tsien, J. Z. (2005). Requirement of NMDA receptor reactivation for consolidation and storage of nondeclarative taste memory revealed by inducible NR1 knockout. Eur. J. Neurosci. 22, 755–763. doi: 10.1111/j.1460-9568.2005.04257.x
Cui, Z., Wang, H., Tan, Y., Zaia, K. A., Zhang, S., and Tsien, J. Z. (2004). Inducible and reversible NR1 knockout reveals crucial role of the NMDA receptor in preserving remote memories in the brain. Neuron 41, 781–793. doi: 10.1016/S0896-6273(04)00072-8
Davis, H. P., and Squire, L. R. (1984). Protein synthesis and memory: a review. Psychol. Bull. 96, 518–519. doi: 10.1037/0033-2909.96.3.518
Drisaldi, B., Colnaghi, L., Fioriti, L., Rao, N., Myers, C., Snyder, A. M., et al. (2015). SUMOylation is an inhibitory constraint that regulates the prion-like aggregation and activity of CPEB3. Cell Rep. 11, 1694–1702. doi: 10.1016/j.celrep.2015.04.061
Eden, E., Geva-Zatorsky, N., Issaeva, I., Cohen, A., Dekel, E., Danon, T., et al. (2011). Proteome half-life dynamics in living human cells. Science 331, 764–768. doi: 10.1126/science.1199784
English, J. D., and Sweatt, J. D. (1997). A requirement for the mitogen-activated protein kinase cascade in hippocampal long-term potentiation. J. Biol. Chem. 272, 19103–19106. doi: 10.1074/jbc.272.31.19103
Fauth, M. J., and van Rossum, M. C. (2019). Self-organized reactivation maintains and reinforces memories despite synaptic turnover. Elife 8:43717. doi: 10.7554/eLife.43717
Fioriti, L., Myers, C., Huang, Y. Y., Li, X., Stephan, J. S., Trifilieff, P., et al. (2015). The persistence of hippocampal-based memory requires protein synthesis mediated by the prion-like protein CPEB3. Neuron 86, 1433–1448. doi: 10.1016/j.neuron.2015.05.021
Fonkeu, Y., Kraynyukova, N., Hafner, A. S., Kochen, L., Sartori, F., Schuman, E. M., et al. (2019). How mRNA localization and protein synthesis sites influence dendritic protein distribution and dynamics. Neuron 103, 1109–1122. doi: 10.1016/j.neuron.2019.06.022
Freeman, J. H. (2015). Cerebellar learning mechanisms. Brain Res. 1621, 260–269. doi: 10.1016/j.brainres.2014.09.062
Frey, U., Krug, M., Reymann, K. G., and Matthies, H. (1998). Anisomycin, an inhibitor of protein synthesis, blocks late phases of LTP phenomena in the hippocampal CA1 region in vitro. Brain Res. 452, 57–65. doi: 10.1016/0006-8993(88)90008-X
Frey, U., and Morris, R. G. (1997). Synaptic tagging and long-term potentiation. Nature 385, 533–536. doi: 10.1038/385533a0
Frey, U., and Morris, R. G. (1998). Synaptic tagging: implications for late maintenance of hippocampal long-term potentiation. Trends Neurosci. 21, 181–188. doi: 10.1016/S0166-2236(97)01189-2
Gridchyn, I., Schoenenberger, P., O'Neill, J., and Csicsvari, J. (2020). Assembly-specific disruption of hippocampal replay leads to selective memory deficit. Neuron 106:291–300. doi: 10.1016/j.neuron.2020.01.021
Hawkins, R. D., and Byrne, J. H. (2015). Associative learning in invertebrates. Cold Spring Harb. Perspect. Biol. 7:a021709. doi: 10.1101/cshperspect.a021709
Helfer, P., and Shultz, T. R. (2018). Coupled feedback loops maintain synaptic long-term potentiation: a computational model of PKMzeta synthesis and AMPA receptor trafficking. PLoS Comput. Biol. 14:e1006147. doi: 10.1371/journal.pcbi.1006147
Herberg, F. W., Taylor, S. S., and Dostmann, W. R. (1996). Active site mutations define the pathway for the cooperative activation of cAMP-dependent protein kinase. Biochemistry 35, 2934–2942. doi: 10.1021/bi951647c
Hernandez, A. I., Blace, N., Crary, J. F., Serrano, P. A., Leitges, M., Libien, J. M., et al. (2003). Protein kinase M synthesis from a brain mRNA encoding an independent protein kinase C catalytic domain. Implications for the molecular mechanism of memory. J. Biol. Chem. 278, 40305–40316. doi: 10.1074/jbc.M307065200
Hige, T. (2018). What can tiny mushrooms in fruit flies tell us about learning and memory? Neurosci. Res. 129, 8–16. doi: 10.1016/j.neures.2017.05.002
Holliday, R. (1999). Is there an epigenetic component in long-term memory? J. Theor. Biol. 200, 339–341. doi: 10.1006/jtbi.1999.0995
Hsieh, C., Tsokas, P., Serrano, P., Hernández, A. I., Tian, D., Cottrell, J. E., et al. (2017). Persistent increased PKMζ in long-term and remote spatial memory. Neurobiol. Learn. Mem. 138, 135–144. doi: 10.1016/j.nlm.2016.07.008
Ikegaya, Y., Aaron, G., Cossart, R., Aronov, D., Lampl, I., Ferster, D., et al. (2004). Synfire chains and cortical songs: temporal modules of cortical activity. Science 304, 559–564. doi: 10.1126/science.1093173
Jalil, S. J., Sacktor, T. C., and Shouval, H. Z. (2015). Atypical PKCs in memory maintenance: the roles of feedback and redundancy. Learn. Mem. 22, 344–353. doi: 10.1101/lm.038844.115
Josselyn, S. A., Kohler, S., and Frankland, P. W. (2017). Heroes of the engram. J. Neurosci. 37, 4647–4657. doi: 10.1523/JNEUROSCI.0056-17.2017
Josselyn, S. A., and Tonegawa, S. (2020). Memory engrams: recalling the past and imagining the future. Science 367:eaaw4325. doi: 10.1126/science.aaw4325
Kandel, E. R. (2001). The molecular biology of memory storage: a dialogue between genes and synapses. Science 294, 1030–1038. doi: 10.1126/science.1067020
Kelly, M. T., Crary, J. F., and Sacktor, T. C. (2007). Regulation of protein kinase M synthesis by multiple kinases in long-term potentiation. J. Neurosci. 27, 3439–3444. doi: 10.1523/JNEUROSCI.5612-06.2007
Ko, H. G., Kim, J., Sim, S. E., Kim, T., Yoo, J., Choi, S. L., et al. (2016). The role of nuclear PKMzeta in memory maintenance. Neurobiol. Learn. Mem. 135, 50–56. doi: 10.1016/j.nlm.2016.06.010
Kolarow, R., Brigadski, T., and Lessmann, V. (2007). Postsynaptic secretion of BDNF and NT-3 from hippocampal neurons depends on calcium calmodulin kinase II signaling and proceeds via delayed fusion pore opening. J. Neurosci. 27, 10350–10364. doi: 10.1523/JNEUROSCI.0692-07.2007
Kovalchuk, Y., Hanse, E., Kafitz, K. W., and Konnerth, A. (2002). Postsynaptic induction of BDNF-mediated long-term potentiation. Science 295, 1729–1734. doi: 10.1126/science.1067766
Kyrke-Smith, M., and Williams, J. M. (2018). Bridging synaptic and epigenetic maintenance mechanisms of the engram. Front. Mol. Neurosci. 11:369. doi: 10.3389/fnmol.2018.00369
Lamsa, K., and Lau, P. (2019). Long-term plasticity of hippocampal interneurons during in vivo memory processes. Curr. Opin. Neurobiol. 54, 20–27. doi: 10.1016/j.conb.2018.08.006
Lee, A. M., Kanter, B. R., Wang, D., Lim, J. P., Zou, M. E., Qiu, C., et al. (2013). Pkrcz null mice show normal learning and memory. Nature 493, 416–419. doi: 10.1038/nature11803
Lee, S. R., Escobedo-Lozoya, Y., Szatmeri, E. M., and Yasuda, R. (2009). Activation of CaMKII in single dendritic spines during long-term potentiation. Nature 458, 299–304. doi: 10.1038/nature07842
Lengyel, I., Voss, K., Cammarota, M., Bradshaw, K., Brent, V., Murphy, K. P., et al. (2004). Autonomous activity of CAMKII is only transiently increased following the induction of long-term potentiation in the rat hippocampus. Eur. J. Neurosci. 20, 3063–3072. doi: 10.1111/j.1460-9568.2004.03748.x
Li, L., McGinnis, J. P., and Si, J. K. (2018). Translational control by prion-like proteins. Trends Cell Biol. 28, 494–505. doi: 10.1016/j.tcb.2018.02.002
Li, Q., Rothkegel, M., Xiao, Z. C., Abraham, W. C., Korte, M., and Sajikumar, S. (2014). Making synapses strong: metaplasticity prolongs associativity of long-term memory by switching synaptic tag mechanisms. Cereb. Cortex 24, 353–363. doi: 10.1093/cercor/bhs315
Ling, D. S., Benardo, L. S., Serrano, P. A., Blace, N., Kelly, M. T., Crary, J. F., et al. (2002). Protein kinase Mzeta is necessary and sufficient for LTP maintenance. Nat. Neurosci. 5, 295–296. doi: 10.1038/nn829
Lisman, J. E. (1985). A mechanism for memory storage insensitive to molecular turnover: a bistable autophosphorylating kinase. Proc. Natl. Acad. Sci. U.S.A. 82, 3055–3057. doi: 10.1073/pnas.82.9.3055
Lisman, J. E., and Goldring, M. A. (1988). Feasibility of long-term storage of graded information by the Ca2+ / calmodulin-dependent protein kinase molecules of the postsynaptic density. Proc. Natl. Acad. Sci. U.S.A. 85, 5320–5324. doi: 10.1073/pnas.85.14.5320
Liu, J., Fukunaga, K., Yamamoto, H., Nishi, K., and Miyamoto, E. (1999). Differential roles of Ca2+/calmodulin-dependent protein kinase II and mitogen-activated protein kinase activation in hippocampal long-term potentiation. J. Neurosci. 19, 8292–8299. doi: 10.1523/JNEUROSCI.19-19-08292.1999
Liu, X., Ramirez, S., Pang, P. T., Puryear, C. B., Govindarajan, A., Deisseroth, K., et al. (2012). Optogenetic stimulation of a hippocampal engram activates fear memory recall. Nature 484, 381–385. doi: 10.1038/nature11028
Lu, Y., Ji, Y., Ganesan, S., Schloesser, R., Martinowich, K., Sun, M., et al. (2011). TrkB as a potential synaptic and behavioral tag. J. Neurosci. 31, 11762–11771. doi: 10.1523/JNEUROSCI.2707-11.2011
Lynch, M. A. (2004). Long-term potentiation and memory. Physiol. Rev. 84, 87–136. doi: 10.1152/physrev.00014.2003
Malenka, R. C., Kauer, J. A., Perkel, D. J., Mauk, M. D., Kelly, P. T., Nicoll, R. A., et al. (1989). An essential role for postsynaptic calmodulin and protein kinase activity in long-term potentiation. Nature 340, 554–557. doi: 10.1038/340554a0
Malenka, R. C., Lancaster, B., and Zucker, R. S. (1992). Temporal limits on the rise in postsynaptic calcium required for the induction of long-term potentiation. Neuron 9, 121–128. doi: 10.1016/0896-6273(92)90227-5
Matsushita, M., Tomizawa, K., Moriwaki, A., Li, S. T., Terada, H., and Matsui, H. (2001). A high efficiency protein transduction system demonstrating the role of PKA in long-lasting long-term potentiation. J. Neurosci. 21, 6000–6007. doi: 10.1523/JNEUROSCI.21-16-06000.2001
Mayford, M., Siegelbaum, S. A., and Kandel, E. R. (2012). Synapses and memory storage. Cold Spring Harb. Perspect. Biol. 4:a005751. doi: 10.1101/cshperspect.a005751
Migues, P. V., Hardt, O., Wu, D. C., Gamache, K., Sacktor, T. C., Wang, Y. T., et al. (2010). PKMzeta maintains memories by regulating GluR2-dependent AMPA receptor trafficking. Nat. Neurosci. 13, 630–634. doi: 10.1038/nn.2531
Miller, J. E., Ayzenshtat, I., Carrillo-Reid, L., and Yuste, R. (2014). Visual stimuli recruit intrinsically generated cortical ensembles. Proc. Natl. Acad. Sci. U.S.A. 111, E4053–E4061. doi: 10.1073/pnas.1406077111
Miller, P., Zhabotinsky, A. M., Lisman, J. E., and Wang, X. J. (2005). The stability of a stochastic CaMKII switch: dependence on the number of enzyme molecules and protein turnover. PLoS Biol. 3:e107. doi: 10.1371/journal.pbio.0030107
Mirisis, A. A., Alexandrescu, A., Carew, T. J., and Kopec, A. M. (2016). The contribution of spatial and temporal molecular networks in the induction of long-term memory and its underlying synaptic plasticity. AIMS Neurosci. 3, 356–384. doi: 10.3934/Neuroscience.2016.3.356
Mozzachiodi, R., and Byrne, J. H. (2010). More than synaptic plasticity: role of nonsynaptic plasticity in learning and memory. Trends Neurosci. 33, 17–26. doi: 10.1016/j.tins.2009.10.001
Murakoshi, H., Shin, M. E., Parra-Bueno, P., Szatmari, E. M., Shibata, A. C. E., and Yasuda, R. (2017). Kinetics of endogenous CaMKII required for synaptic plasticity revealed by optogenetic kinase inhibitor. Neuron 94, 37–47. doi: 10.1016/j.neuron.2017.02.036
Nabavi, S., Fox, R., Proulx, C. D., Lin, J. Y., Tsien, R. Y., and Malinow, R. (2014). Engineering a memory with LTD and LTP. Nature 511, 348–352. doi: 10.1038/nature13294
Otmakhov, N., Griffith, L. C., and Lisman, J. E. (1997). Postsynaptic inhibitors of calcium/calmodulin-dependent protein kinase type II block induction but not maintenance of pairing-induced long-term potentiation. J. Neurosci. 17, 5357–5365. doi: 10.1523/JNEUROSCI.17-14-05357.1997
Panja, D., and Bramham, C. R. (2014). BDNF mechanisms in late LTP formation: synthesis and breakdown. Neuropharmacology 76, 664–676. doi: 10.1016/j.neuropharm.2013.06.024
Park, S., Kramer, E. E., Mercaldo, V., Rashid, A. J., Insel, N., Frankland, P. W., et al. (2016). Neuronal allocation to a hippocampal engram. Neuropsychopharmacology 41, 2987–2993. doi: 10.1038/npp.2016.73
Pastalkova, E., Serrano, P., Pinkhasova, D., Wallace, E., Fenton, A. A., and Sacktor, T. C. (2006). Storage of spatial information by the maintenance mechanism of LTP. Science 313, 1141–1144. doi: 10.1126/science.1128657
Pettigrew, D. B., Smolen, P., Baxter, D. A., and Byrne, J. H. (2005). Dynamic properties of regulatory motifs associated with induction of three temporal domains of memory in Aplysia. J. Comput. Neurosci. 18, 163–181. doi: 10.1007/s10827-005-6557-0
Pologruto, T. A., Yasuda, R., and Svoboda, K. (2004). Monitoring neural activity and [Ca2+] with genetically encoded Ca2+ indicators. J. Neurosci. 24, 9572–9579. doi: 10.1523/JNEUROSCI.2854-04.2004
Ramachandran, B., and Frey, J. U. (2009). Interfering with the actin network and its effect on long-term potentiation and synaptic tagging in hippocampal CA1 neurons in slices in vitro. J. Neurosci. 29, 12167–12173. doi: 10.1523/JNEUROSCI.2045-09.2009
Rayman, J. B., and Kandel, E. R. (2017). Functional prions in the brain. Cold Spring Harb. Perspect. Biol. 9:a023671. doi: 10.1101/cshperspect.a023671
Redondo, R. L., Okuno, H., Spooner, P. A., Frenguelli, B. G., Bito, H., and Morris, R. G. (2010). Synaptic tagging and capture: differential role of distinct calcium/calmodulin kinases in protein synthesis-dependent long-term potentiation. J. Neurosci. 30, 4981–4989. doi: 10.1523/JNEUROSCI.3140-09.2010
Roberson, E. D., and Sweatt, J. D. (1996). Transient activation of cyclic AMP-dependent protein kinase during hippocampal long-term potentiation. J. Biol. Chem. 271, 30436–30441. doi: 10.1074/jbc.271.48.30436
Roberson, E. D., and Sweatt, J. D. (1999). A biochemical blueprint for long-term memory. Learn. Mem. 6, 381–388.
Rosenblum, K., Futter, M., Voss, K., Erent, M., Skehel, P. A., French, P., et al. (2002). The role of extracellular regulated kinases I/II in late-phase long-term potentiation. J. Neurosci. 22, 5432–5441. doi: 10.1523/JNEUROSCI.22-13-05432.2002
Rossetti, T., Banerjee, S., Kim, C., Leubner, M., Lamar, C., Gupta, P., et al. (2017). Memory erasure experiments indicate a critical role of CaMKII in memory storage. Neuron 96, 207–216. doi: 10.1016/j.neuron.2017.09.010
Ryan, T. J., Roy, D. S., Pignatelli, M., Arons, A., and Tonegawa, S. (2015). Engram cells retain memory under retrograde amnesia. Science 348, 1007–1013. doi: 10.1126/science.aaa5542
Sacktor, T. C., Osten, P., Valsamis, H., Jiang, X., Naik, M. U., and Sublette, E. (1993). Persistent activation of the zeta isoform of protein kinase C in the maintenance of long-term potentiation. Proc. Natl. Acad. Sci. U.S.A. 90, 8342–8346. doi: 10.1073/pnas.90.18.8342
Saneyoshi, T., Matsuno, H., Suzuki, A., Murakoshi, H., Hedrick, N. G., Agnello, E., et al. (2019). Reciprocal activation within a kinase-effector complex underlying persistence of structural LTP. Neuron 102, 1199–1210. doi: 10.1016/j.neuron.2019.04.012
Sanhueza, M., McIntyre, C. C., and Lisman, J. E. (2007). Reversal of synaptic memory by a Ca2+ / calmodulin-dependent protein kinase II inhibitor. J. Neurosci. 27, 5190–5199. doi: 10.1523/JNEUROSCI.5049-06.2007
Schapiro, A. C., McDevitt, E. A., Rogers, T. T., Mednick, S. C., and Norman, K. A. (2018). Human hippocampal replay during rest prioritizes weakly learned information and predicts memory performance. Nat. Commun. 9:3920. doi: 10.1038/s41467-018-06213-1
Schwartz, J. H. (1993). Cognitive kinases. Proc. Natl. Acad. Sci. U.S.A. 90, 8310–8313. doi: 10.1073/pnas.90.18.8310
Serrano, P., Friedman, E. L., Kenney, J., Taubenfeld, S. M., Zimmerman, J. M., Hanna, J., et al. (2008). PKMzeta maintains spatial, instrumental, and classically conditioned long-term memories. PloS Biol. 6, 2698–2706. doi: 10.1371/journal.pbio.0060318
Serrano, P., Yao, Y., and Sacktor, T. C. (2005). Persistent phosphorylation by protein kinase Mzeta maintains late-phase long-term potentiation. J. Neurosci. 25, 1979–1984. doi: 10.1523/JNEUROSCI.5132-04.2005
Shema, R., Haramati, S., Ron, S., Hazvi, S., Chen, A., Sacktor, T. C., et al. (2011). Enhancement of consolidated long-term memory by overexpression of protein kinase Mzeta in the neocortex. Science 331, 1207–1210. doi: 10.1126/science.1200215
Shema, R., Sacktor, T. C., and Dudai, Y. (2007). Rapid erasure of long-term memory associations in the cortex by an inhibitor of PKM zeta. Science 317, 951–953. doi: 10.1126/science.1144334
Shimizu, E., Tang, Y. P., Rampon, C., and Tsien, J. Z. (2000). NMDA receptor-dependent synaptic reinforcement as a crucial process for memory consolidation. Science 290, 1170–1174. doi: 10.1126/science.290.5494.1170
Si, K., Choi, Y. B., White-Grindley, E., Majumdar, A., and Kandel, E. R. (2010). Aplysia CPEB can form prion-like multimers in sensory neurons that contribute to long-term facilitation. Cell 140, 421–435. doi: 10.1016/j.cell.2010.01.008
Si, K., Giustetto, M., Etkin, A., Hsu, R., Janisiewicz, A. M., Miniaci, M. C., et al. (2003). A neuronal isoform of CPEB regulates local protein synthesis and stabilizes synapse-specific long-term facilitation in Aplysia. Cell 115, 893–904. doi: 10.1016/S0092-8674(03)01021-3
Singh, D., and Bhalla, U. S. (2018). Subunit exchange enhances information retention by CaMKII in dendritic spines. Elife 7:e41412. doi: 10.7554/eLife.41412
Smolen, P. (2007) A model of late long-term potentiation simulates aspects of memory maintenance. PLoS One 2, e445. doi: 10.1371/journal.pone.0000445.
Smolen, P., Baxter, D. A., and Byrne, J. H. (2006). A model of the roles of essential kinases in the induction and expression of late long-term potentiation. Biophys. J. 90, 2760–2775. doi: 10.1529/biophysj.105.072470
Smolen, P., Baxter, D. A., and Byrne, J. H. (2012). Molecular constraints on synaptic tagging and maintenance of long-term potentiation: a predictive model. PLoS Comput. Biol. 8:e1002620. doi: 10.1371/journal.pcbi.1002620
Smolen, P., Baxter, D. A., and Byrne, J. H. (2014). Simulations suggest pharmacological methods for rescuing long-term potentiation. J. Theor. Biol. 360, 243–250. doi: 10.1016/j.jtbi.2014.07.006
Smolen, P., Baxter, D. A., and Byrne, J. H. (2019). How can memories last for days, years, or a lifetime? Proposed mechanisms for maintaining synaptic potentiation and memory. Learn. Mem. 26, 133–150. doi: 10.1101/lm.049395.119
Song, H., Smolen, P., Av-Ron, E., Baxter, D. A., and Byrne, J. H. (2007). Dynamics of a minimal model of interlocked positive and negative feedback loops of transcriptional regulation by cAMP-response element binding proteins. Biophys J. 92, 3407–3424. doi: 10.1529/biophysj.106.096891
Stanton, P. K., and Sarvey, J. M. (1984). Blockade of long-term potentiation in rat hippocampal CA1 region by inhibitors of protein synthesis. J. Neurosci. 4, 3080–3088. doi: 10.1523/JNEUROSCI.04-12-03080.1984
Tonegawa, S., Morrissey, M. D., and Kitamura, T. (2018). The role of engram cells in the systems consolidation of memory. Nat. Rev. Neurosci. 19, 485–498. doi: 10.1038/s41583-018-0031-2
Turrigiano, G. G., Leslie, K. R., Desai, N. S., Rutherford, L. C., and Nelson, S. B. (1998). Activity-dependent scaling of quantal amplitude in neocortical neurons. Nature 391, 892–896. doi: 10.1038/36103
Vanyushin, B. F., Tushmalova, N. A., and Guskova, L. V. (1974). Brain DNA methylation as an index of genome participation in mechanism of memory formation. Doklady Akademii Nauk SSSR 219, 742–744.
Vincent, P., and Brusciano, D. (2001). Cyclic AMP imaging in neurons in brain slice preparation. J. Neurosci. Method 108, 189–198. doi: 10.1016/S0165-0270(01)00393-4
Volk, L. J., Bachman, J. L., Johnson, R., Yu, Y., and Huganir, R. L. (2013). PKM-ζ is not required for hippocampal synaptic plasticity, learning and memory. Nature 493, 420–423. doi: 10.1038/nature11802
Wang, S., Sheng, T., Ren, S., Tian, T., and Lu, W. (2016). Distinct roles of PKMζ and PKCι/λ in the initiation and maintenance of hippocampal long-term potentiation and memory. Cell Rep. 16, 1954–1961. doi: 10.1016/j.celrep.2016.07.030
Westmark, P. R., Westmark, C. J., Wang, S., Levenson, J., O'Riordan, K. J., Burger, C., et al. (2010). Pin1 and PKMzeta sequentially control dendritic protein synthesis. Sci. Signal. 3:ra18. doi: 10.1126/scisignal.2000451
Woo, N. H., Duffy, S. N., Abel, T., and Nguyen, P. V. (2000). Genetic and pharmacological demonstration of differential recruitment of cAMP-dependent protein kinases by synaptic activity. J. Neurophysiol. 84, 2739–2745. doi: 10.1152/jn.2000.84.6.2739
Wu, X., and Foster, D. J. (2014). Hippocampal replay captures the unique topological structure of a novel environment. J. Neurosci. 34, 6459–6469. doi: 10.1523/JNEUROSCI.3414-13.2014
Ying, S. W., Futter, M., Rosenblum, K., Webber, M. J., Hunt, S. P., Bliss, T. V. P., et al. (2002). Brain-derived neurotrophic factor induces long-term potentiation in intact adult hippocampus: requirement for ERK activation coupled to CREB and upregulation of Arc synthesis. J. Neurosci. 22, 1532–1540. doi: 10.1523/JNEUROSCI.22-05-01532.2002
Zhabotinsky, A. M. (2000). Bistability in the Ca(2+)/calmodulin-dependent protein kinase-phosphatase system. Biophys. J. 79, 2211–2221. doi: 10.1016/S0006-3495(00)76469-1
Zhang, Y., Smolen, P., Alberini, C. M., Baxter, D. A., and Byrne, J. H. (2016). Computational model of a positive BDNF feedback loop in hippocampal neurons following inhibitory avoidance training. Learn. Mem. 23, 714–722. doi: 10.1101/lm.042044.116
Zhang, Y., Smolen, P., Baxter, D. A., and Byrne, J. H. (2010). The sensitivity of memory consolidation and reconsolidation to inhibitors of protein synthesis and kinases: computational analysis. Learn. Mem. 17, 428–439. doi: 10.1101/lm.1844010
Keywords: memory, long-term potentiation, engram, replay, model, computational
Citation: Smolen P, Baxter DA and Byrne JH (2020) Comparing Theories for the Maintenance of Late LTP and Long-Term Memory: Computational Analysis of the Roles of Kinase Feedback Pathways and Synaptic Reactivation. Front. Comput. Neurosci. 14:569349. doi: 10.3389/fncom.2020.569349
Received: 03 June 2020; Accepted: 16 November 2020;
Published: 16 December 2020.
Edited by:
Nicolas Brunel, Duke University, United StatesReviewed by:
Michael Graupner, New York University, United StatesJeanette Hellgren Kotaleski, Karolinska Institutet (KI), Sweden
Copyright © 2020 Smolen, Baxter and Byrne. This is an open-access article distributed under the terms of the Creative Commons Attribution License (CC BY). The use, distribution or reproduction in other forums is permitted, provided the original author(s) and the copyright owner(s) are credited and that the original publication in this journal is cited, in accordance with accepted academic practice. No use, distribution or reproduction is permitted which does not comply with these terms.
*Correspondence: Paul Smolen, paul.d.smolen@uth.tmc.edu