- 1College of Plant Protection, Technological Innovation Center for Biological Control of Crop Diseases and Insect Pests of Hebei Province, Hebei Agricultural University, Baoding, China
- 2College of Biological Sciences and Engineering, Hebei Xingtai College, Xingtai, China
- 3Graduate School of Chinese Academy of Agricultural Sciences, Beijing, China
Wheat leaf rust caused by Puccinia triticina is one of the most common and serious diseases in wheat production. The constantly changing pathogens overcome the plant resistance to P. triticina. Plant pathogens secrete effector proteins that alter the structure of the host cell, interfere plant defenses, or modify the physiology of plant cells. Therefore, the identification of effector proteins is critical to reveal the pathogenic mechanism. We used SignalP v4.1, TargetP v1.1, TMHMM v2.0, and EffectorP v2.0 to screen the candidate effector proteins in P. triticina isolates – KHTT, JHKT, and THSN. As a result, a total of 635 candidate effector proteins were obtained. Structural analysis showed that effector proteins were small in size (50AA to 422AA) and of diverse sequences, and the conserved sequential elements or clear common elements were not involved, regardless of their secretion from the pathogen to the host. There were 427 candidate effector proteins that contain more than or equal to 4 cysteine residues, and 339 candidate effector proteins contained the known motifs. Sixteen families, 9 domains, and 53 other known functional types were found in 186 candidate effector proteins using the Pfam search. Three novel motifs were found by MEME. Heterogeneous expression system was performed to verify the functions of 30 candidate effectors by inhibiting the programmed cell death (PCD) induced by BAX (the mouse-apoptotic gene elicitor) on Nicotiana benthamiana. Hypersensitive response (HR) can be induced by the six effectors in the wheat leaf rust resistance near isogenic lines, and this would be shown by the method of transient expression through Agrobacterium tumefaciens infiltration. The quantitative reverse transcription PCR (qRT-PCR) analysis of 14 candidate effector proteins secreted after P. triticina inoculation showed that the tested effectors displayed different expression patterns in different stages, suggesting that they may be involved in the wheat–P. triticina interaction. The results showed that the prediction of P. triticina effector proteins based on transcriptomic analysis and multiple bioinformatics software is effective and more accurate, laying the foundation of revealing the pathogenic mechanism of Pt and controlling disease.
Introduction
Wheat leaf rust caused by Puccinia triticina is an airborne, destructive, and obligate biotrophic fungal diseases. Epidemic of this disease can cause 15–45% losses of production and threaten food security worldwide. Plant – pathogen interaction is regulated by multilayers immune mechanism, which can be activated through recognition of pathogens by host-resistant genes. Pathogens interfere with the host defensive identification signal network by secreting effector proteins that reprogram plant cell structure or metabolism (Kamoun, 2006; Lo Presti et al., 2015). The fungal effector proteins can be attached to the cell wall to act in plant apoplast or be transferred to the plant cells targeting specific host proteins or entering corresponding cell tissues (Lo Presti et al., 2015). Understanding the mechanisms of different pathogenic effector proteins can reveal molecular mechanisms of pathogenicity and develop sustainable control strategies for crops (Dalio et al., 2017).
To date, only a few pathogenic fungal effectors have been obtained, and their functions have been understood. However, with the development of whole genome sequencing and the increased numbers of pathogen genomes, complex computational methods have been developed to predict candidate secreted effector proteins (CSEPs) in the pathogen genomes (Sperschneider et al., 2015). Predicting the CSEPs suggests that it may be located extracellularly or belong to the secreted proteins. Many studies have shown that the avirulent genes or effectors encoded by pathogenicity-related genes are secreted proteins. For example, the encoded product of the flax rust fungal AvrL567 (Dodds et al., 2004; Catanzariti et al., 2006), Avr1b of Phytophthora sojae (Shan et al., 2004), the avirulence genes of rice blast fungus Avr Pita and Pwl2 (Sweigard et al., 1995), the proteins encoded by AvrSr35 (Salcedo et al., 2017) and AvrSr50 (Chen et al., 2017) of Puccinia gramminis f. sp. tritici are secreted proteins. Numerous researches have shown that elicitors and pathogenic factors secreted out from the pathogen, which can be recognized by plant receptor proteins (D’Silva and Heath, 1997). The role of the signal peptide is to direct the proteins from the cell to the endoplasmic reticulum. It is cleaved by cotranslation, and the mature protein is released from the cell by the Golgi during the secretion (Dalio et al., 2017). Therefore, signal peptide play an extremely important role in the transport of CSEPs and the recognition of plant receptor proteins (Catanzariti et al., 2006). These products encoded by avirulence genes with signal peptide may be involved in the process of information transfer after recognizing plant elicitor receptors (Han et al., 2005). Based on a comprehensive analysis of existing effectors, it was found that most of the CSEPs have the following characteristics, such as containing signal peptide (SP), no transmembrane domain, and small molecular weight (5–30 kDa). In addition, analysis is based on a number of secondary features, such as effector protein motifs, nuclear localization signals, genomic localization, and three-dimensional structures. Based on the characteristics of these effector proteins, models and software have been developed for predicting CSEPs. SignalP (Bendtsen et al., 2004) based on the combination of several artificial neural networks is capable of predicting signal peptide/non-signal peptide; the presence and location of the SP site in the amino acid sequence are different in different organisms, such as Gram-positive/Gram-negative prokaryotes and eukaryotes. TargetP (Emanuelsson et al., 2000) is reported for the prediction of gene localization and is capable of distinguishing chloroplast transit peptide (cTP), mitochondrial transit peptide (mTP), and signal peptide (SP) with higher sensitivity and specificity than any other available subcellular localization prediction software. It has of relatively good capability of the site prediction for relevant target sequences. Predicting the location and the number of transmembrane helices is very important for membrane proteins. TMHMM (Krogh et al., 2001) established by the hidden Markov model has been built to specifically model the variable protein regions of membrane proteins. The hydrophobicity, charge bias, and helix length are combined into one model through setting the upper and lower limits of membrane helix length to predict membrane proteins and to count the different amounts of topologies. Up to now, bioinformatics analysis has been applied to predict fungal effectors in Fusarium graminearum, Puccinia striiformis f. sp. tritici, etc. (Prasad et al., 2019).
Recent progress in genomics results in many high-quality P. triticina genomes and gene expression profiles during wheat infection by P. triticina (Bruce et al., 2014; Cuomo et al., 2017). The expression profiles of secreted proteins during the interaction between wheat and P. triticina are typically complicated. There are many secreted non-effectors that play roles in colonization and protection of P. triticina from competing microbes, differentiation of fungal structures, and cell-to-cell communication. Therefore, accurate mining of effector proteins is time saving for subsequent experimental validation. Using machine learning approach to predict effector proteins is the most convenient and effective way (Sperschneider et al., 2018a).
EffectorP developed based on the Bayesian classification can improve the prediction efficiency and precisely screen the effectors secreted by pathogenic fungi according to the molecular weight, the net charge, and the percentage of cysteine, serine, and tryptophan of the protein (Sperschneider et al., 2016).
Transcriptome analysis is conducive to identify the CSEPs and provide a tool for studying the interaction between rust and host and discovering the pathogenesis of rust. Because of the large genome and complex structure of rust, RNA-seq is an effective method for the CSEPs’ identification. At present, this approach has been applied to different species and genera of rust.
P. triticina is a dikaryotic fungus in the most time of life cycle. The genome of P. triticina is around 135 MB, which is the largest one among P. gramminis f. sp. tritici, P. striiformis f. sp. tritici, and P. triticina. A total of 1,358 secreted proteins were predicted based on the genome and RNA-seq of P. triticina isolate – BBBD. There were 784 candidate effector proteins expressed in diverse stages of life cycle including the sexual stage (Cuomo et al., 2017). Different pathogenic types exhibit different CSEPs when interacting with the host. We have carried out RNA-seq for three different P. triticina pathotypes (THSN, JHKT, and KHHT) at the stage of dormant urediniospores, germinated urediniospores, and interaction with wheat cultivar “Thatcher” at 6 days post-inoculation (dpi).
In this study, we used many software including SignalP v4.1, TargetP v1.1, TMHMM v2.0, and EffectorP v2.0 to predict the effector proteins based on RNA-seq data from three P. triticina isolates at three different stages. The candidate effector proteins were verified by A. tumefaciens-mediated transient expression assay and qRT-PCR. The results will lay a foundation for further study on effector proteins and their pathogenesis of P. triticina.
Materials and Methods
Plant Materials and Fungal Isolates
Wheat cultivars Thatcher, 36 near-isogenic lines or cultivars [TcLr1, TcLr2a, TcLr2b, TcLr2c, TcLr3, TcLr3bg, TcLr3ka, TcLr9, TcLr10, TcLr11, TcLr14a, TcLr14b, TcLr15, TcLr16, TcLr17, TcLr18, TcLr19, TcLr21, TcLr23, TcLr24, TcLr25, TcLr26, TcLr28, TcLr29, TcLr30, TcLr32, TcLr33, TcLr36, TcLr38, TcLr39, KS91WGRC11 (Lr42), TcLr44, TcLr45, Pavin76 (Lr46), TcLr47, TcLr50] and Zhengzhou 5389, P. triticina isolate THSN, which is compatible interacted with “Thatcher” and Zhengzhou 5389, and N. benthamiana were used in this study. Wheat and N. benthamiana plants were grown in the greenhouse at 23 and 16°C, respectively. The THSN was inoculated on the wheat cultivar Zhengzhou 5389 and incubated in the dark at 20°C with 100% relative humidity for 14 h. Plants were then transferred to a greenhouse with 16/8 h day/night cycle at 23–25°C.
RNA Preparation and RNA-Seq
The P. triticina pathotypes were purified by isolating single uredinium and propagated in Zhengzhou 5389, and then, the total RNA of P. triticina at dormant urediniospores, germinated urediniospores, and the same position of the inoculated “Thatcher” leaf tissue by P. triticina isolates KHTT, JHKT, and THSN at 6 dpi were isolated using RNA extraction kit (RNeasy FFPE Kit, Qiagen) following the instructions, respectively (with two or three biological replicates). The Illumina HiSeq 2000 sequencing platform was used for sequencing total RNA after testing the quality of the extracted RNA (conducted by BGI Company, China).
Plasmid Construction and Preparation
The primers used for plasmid construction are shown in Supplementary Table S1. Primers were designed based on the complementary DNA (cDNA) sequence containing the longest open reading frame (ORF) of the CSEPs. Thirty candidate genes screened from the RNA-seq data were PCR amplified and cloned using FastPfu DNA polymerase (TransGen Biotech, Beijing, China). To express the candidate effector genes in N. benthamiana, the sequences encoding mature proteins without the putative signal peptide (SP) were recombined into the PVX vector pGR107. A. tumefaciens strain GV3101 cultured in Luria–Bertani (LB) media containing 50 mg/ml of rifampicin at 28°C with shaking at 220 rpm for 48 h was used for transient expression in N. benthamiana. To detect the expression levels of genes, all primers (Supplementary Table S2) were designed using Primer 5 based on the open reading frame from the RNA-seq.
The Identification of the Coding Sequences
Clusters and unigenes from the RNA-seq data of P. triticina isolates (KHTT, JHKT, and THSN) were analyzed using TransDecoder software to identify the candidate coding sequence (CDS). The homologous sequences predicted by Pfam were searched by Blast alignment SwissProt database and Hmmscan. Then, the CDS were used to predict one by one according to the order of SignalP v4.1, TargetP v1.1, TMHMM v2.0, and EffectorP v2.0 (Supplementary Figure S1).
Signal Peptide Prediction
SP cleavage site prediction was performed on the CDS sequences using the SignalP v4.1 server1. Sequence submission and prediction approaches were based on the description (Nielsen, 2017). The results showing “YES” of D score, which mean the protein contains an N-terminal SP sequence, were selected.
Subcellular Localization Prediction
A new fasta file containing the sequences predicted by SignalP v4.1 was generated and input to the TargetP v1.1 server2 for cell localization prediction. Inputting sequences were according to the instruction (Emanuelsson et al., 2000). The credibility partition is determined by the RC value, which is the difference score (diff) of two consecutive highest values; the higher the RC value level is, the lower the confidence of the data is. This study retained data located at “S” (containing SP) with RC values of 1, 2, and 3, and “M” (containing mitochondrial transit peptide mTP) with RC values of 4 and 5 for predicting protein-containing signal peptide sequences and secreting them extracellularly.
Transmembrane Domain Prediction
A new fasta file containing the sequences predicted by SignalP v4.1 and TargetP v1.1 was generated and submitted to the TMHMM v2.0 (Möller et al., 2001) using the website3. The results showing ExpAAs < 18 and the number of transmembrane structures (PredHel) was equal to “0” were selected for subsequent analysis.
Candidate Effector Proteins Prediction
The selected sequences were extracted and submitted to the EffectorP v2.0 (Sperschneider et al., 2018a)4. The server is based on the molecular weight, the net charge number, and the character of contents of cysteine, serine, and tryptophan in the amino acid of the sequences. The results were displayed in text format; then, the “effector” that is the CSEP was chosen.
Structural Characteristics Analysis of CSEPs
The number of cysteine residues and the size of the amino acids of the CSEPs were counted. Known motifs including RxLR in oomycetes, [Y/F/W]xC in powdery mildew, G[I/F/Y][A/L/S/T]R in flax rust, and [L/I]xAR, [R/K]CxxCx12H, and YxSL[R/K] in Magnaporthe oryzae were searched by Perl scripts. Other known rust effectors were also used to predict the homology with CSEPs. Conservative domain search was performed by Pfam. The candidate effector proteins containing no conserved structures were searched by MEME software.
CSEPs Verification Conducted by BAX-Triggered Suppression Assays on N. benthamiana
The recombinant constructs with CSEPs and the PVX vector pGR107 were introduced into A. tumefaciens strain GV3101 by using the freeze–thaw method. GV3101 containing the corresponding recombinant constructs were cultured in LB media containing 50 mg/ml of kanamycin and 50 mg/ml rifampicin at 28°C with 220 rpm for 48 h. The bacterial pellets were harvested and washed three times with the induction buffer (10 mM MES, 10 mM MgCl2, 400 μM acetosyringone). To achieve an optical density of OD600 = 0.3 by buffer after resuspension, the 6-week-old leaves of N. benthamiana was infiltrated with a needleless syringe. To observe the suppression of cell death induced by BAX, A. tumefaciens cells carrying CSEPs were infiltrated initially, and BAX carrying A. tumefaciens cells were infiltrated at the same site after 24 h. The symptoms were monitored, and photos were taken after 5 days. A. tumefaciens cells carrying enhanced green fluorescent protein (eGFP) was infiltrated and used as negative control. Each assay was repeated on at least three leaves on three plants.
Transient Expression Assay by A. tumefaciens on Wheat Near-Isogenic Lines
According to the method of BAX-triggered suppression assays, using the same control, the bacterial suspension with the optical density of OD600 = 1.2 was infiltrated on the fully expanded secondary leaf of wheat near-isogenic lines with a needleless syringe. The border of the infiltration area was marked with a mark pen. The inoculated leaves were photographed at 7 dpi. The assay for each gene was repeated at least twice, and each repeat consisted of 10 biological replicates.
qRT-PCR Analyze the Expression Level of CSEPs
The total RNA of THSN-inoculated wheat leaves with P. triticina at 0, 6, 12, 18, 24, 36, 48, 60, 72, 96, and 120 h post-inoculation (hpi) were extracted using Plant RNA Extraction Kit (TaKaRa, Japan) following the manufacturer’s instructions. RNA was assessed by agarose gel electrophoresis and quantified by spectrophotometry. About 2.0 μg of each sample was reverse transcribed into cDNA with the 5 × All-In-One RT MasterMix (abm, Jiangsu, China). qRT-PCR reactions were run on a LightCycler detection system using SYBR Green PCR Master Mix (TransGen Biotech, Beijing, China). Elongation factor 1 (EF1) gene was used as the endogenous reference for calculating relative transcription levels. The qRT-PCR thermal profile was set as 5 min at 94°C; 40 cycles of 10 s at 94°C, 15 s at 60°C, and 20 s at 72°C; 1 cycle of 10 s at 95°C, 60 s at 65°C, and 1 s at 97°C; and a final step of 30 s at 37°C. Relative transcript levels of the test gene were calculated by the comparative threshold (2–ΔΔCT) method (Livak and Schmittgen, 2001). Three technical and biological replicates of each treatment were analyzed. Standard deviation was calculated in MS Excel.
Results
RNA-Seq Library and Comprehensive Prediction of CSEPs
We used the company platform to establish RNA-seq library. We generated about 25.81 Gb bases in total on Illumina HiSeq sequencing platform. The total numbers of Unigenes obtained from the databases for KHTT, JHKT, and THSN were 189,266 after assembly and de-redundancy using IIIumina HiSeq platform. A total of 39,741 were complete, and the longest CDS were extracted through Transdecoder software prediction for CSEPs prediction. All raw data were uploaded to National Center for Biotechnology information (NCBI) in BioProject PRJNA6094055.
As a result, a total of 7,158 sequences containing SP were screened out of 39,741 sequences using SignalP v4.1, D score between 0.45 and 0.97, accounting for 18% of the submitted sequences through the NN neural network and hidden Markov model method. Most of the sites of SP were located in 20–30 amino acids. Then, the sequences containing the SP were analyzed using TargetP v1.1. As a result, the locations of genes were shown as “S,” and the RC values of 1, 2, and 3 were 3,050, 1,689, and 716, respectively. In addition, there were 74 and 148 genes located in mitochondrial “M” with RC values of 4 and 5, respectively. A total of 5,677 sequences were obtained for the transmembrane domain prediction by TMHMM v2.0, and 4,514 genes were identified that met ExpAAs < 18 conditions and contained no transmembrane domain.
The 4,514 amino acid sequences were further analyzed by EffectorP v2.0, and a total of 635 sequences were obtained as “Effector,” probabilities of which ranged from 55 to 99% based on amino acid size, molecular weight, sequence length, net protein charge, the hydrophilicity, hydrophobicity, and surface tension of the proteins.
CSEPs of P. triticina Have Typical Structural Characteristics
Statistical analysis of cysteine residues of 635 CSEPs revealed that only 45 effector proteins contained no cysteine and 47 genes contained one cysteine. There are 66, 50, and 80 CSEPs containing 2, 3, and 4 cysteines, respectively. The remaining 347 candidate effector proteins had more than 4 cysteine residues, of which Pt44738 contained 28 cysteines. Amino acid quantity statistics were performed on all CSEPs, and 625 candidate effector proteins were found to be < 300 amino acids. However, 10 genes were >300 amino acids, and the largest size is 422 amino acids.
Using the Perl script to search the known motif, a total of five motifs were found (Figure 1). There are 244 CSEPs that have the “[Y/F/W]xC” motif, the same as in powdery mildew, accounting for 38.43% of the CSEPs in our research. The numbers are 24, 5, 64, and 2 for RxLR, G[I/F/Y][A/L/S/T]R, [L/I]xAR, and YxSL[R/K] motif, respectively. However, there are unknown motifs in 296 CSEPs.
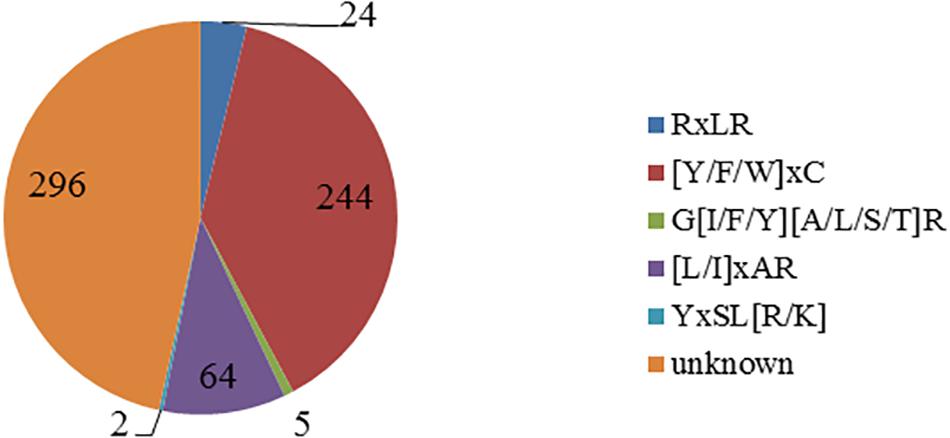
Figure 1. Distribution of the known motifs of 635 candidate effectors. The figure shows five known motifs and quantities.
A conserved domain searching was carried out through Pfam. The results revealed that there are 16 families, 9 domains (Table 1), and 53 other types with known functions (Supplementary Figure S2).
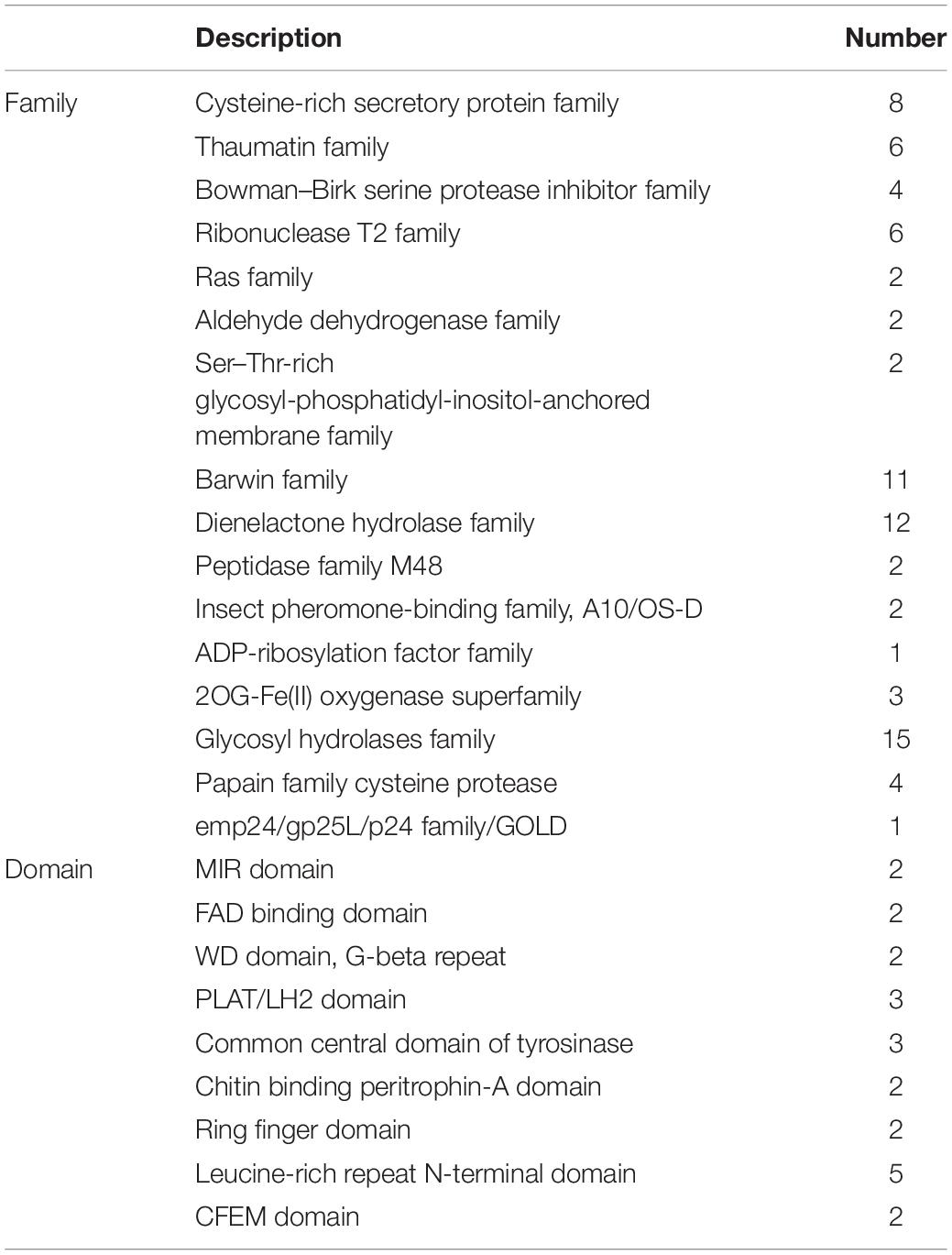
Table 1. Types and quantities of conserved domains of the 635 candidate secreted effector proteins (CSEPs).
The motifs of 296 CSEPs with no conserved structure were searched by MEME. Three motifs with higher E value were found (Figure 2), and motifs 1–3 were present in 16, 15, and 10 CSEPs, respectively. Among them, nine CSEPs contained both motifs 1 and 2. Moreover, Pt53341 contained three motifs (Figure 3).
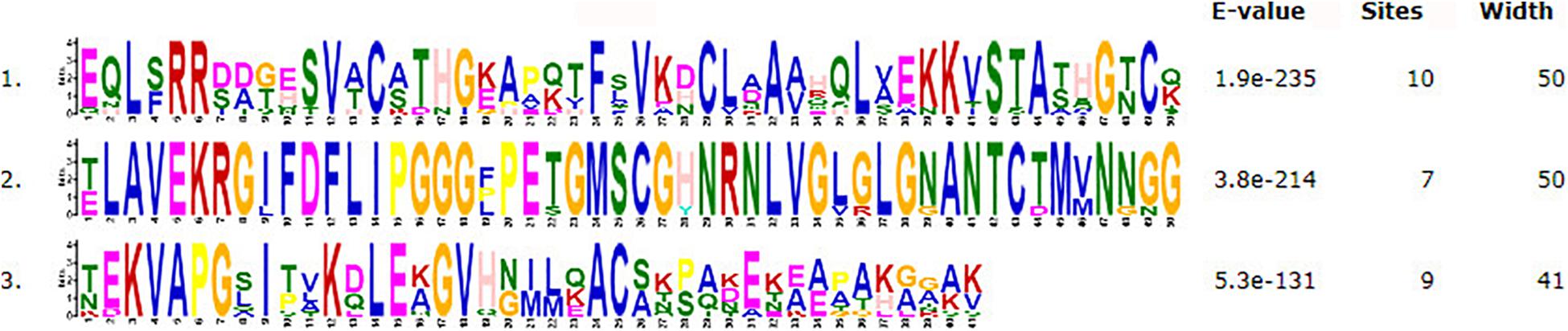
Figure 2. The prediction of three new motifs of candidate secreted effector proteins (CSEPs) by MEME.
Correlation Analysis Between P. triticina CSEPs and Other Rust Sequences
The 635 P. triticina CSEPs were compared with previously reported CSEPs of P. triticina, P. striiformis f. sp. tritici, and P. gramminis f. sp. tritici (Cantu et al., 2013) using TBtools v0.66839 (Chen et al., 2018). We found that 339 sequences share identity with genes in P. triticina. A total of 204 CSEPs have 100% identity with the sequences in BBBD (P. triticina), and the similarities of the remaining CSEPs with BBBD (P. triticina) were more than 70%. Ninety CSEPs were more than 70% similar to that in P. gramminis f. sp. tritici. The identity between Pt20856 and PGTG_024280 was 94%. Fifty-four CSEPs shared sequence similarity with P. striiformis f. sp. tritici, and the identity of Pt72634 with PST43_01110 was 90%. We also found that 20 genes of 635 CSEPs were able to align simultaneously to known effector proteins in P. triticina, P. striiformis f. sp. tritici, and P. gramminis f. sp. tritici with 70% identity (Table 2).
Tested CSEPs Successfully Suppressed PCD Induced by BAX on N. benthamiana
We determined the function of randomly selected 30 CSEPs (Table 3) using an A. tumefaciens-mediated transient expression assay in N. benthamiana (Figure 4). The phenotypes were observed 5 days post-BAX infiltration. The infiltration of the control bacteria suspension carrying pGR107-eGFP did not result in PCD, and the sites infiltrated with pGR107-eGFP and pGR107-BAX exhibited obvious cell death on N. benthamiana. However, the infiltration with pGR107-CSEPs successfully suppressed PCD induced by BAX (Supplementary Figure S3).
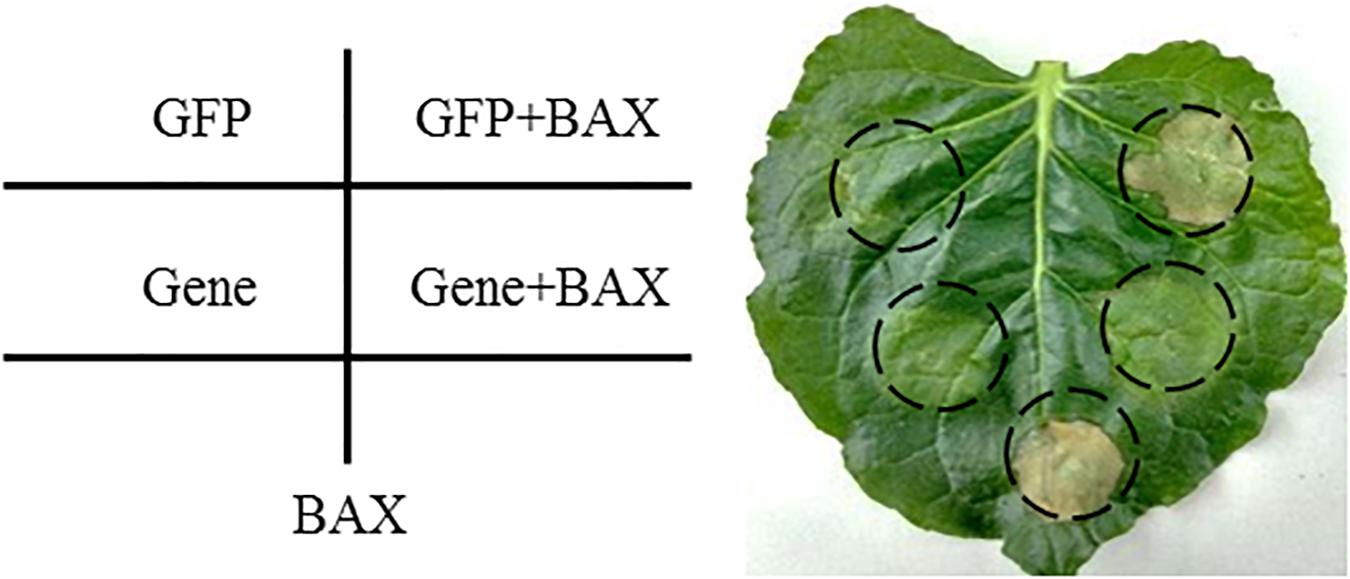
Figure 4. Transient expression of candidate secreted effector proteins (CSEPs) in N. benthamiana suppressed programmed cell death triggered by BAX schematic. N. benthamiana leaves were infiltrated with A. tumefaciens cells containing PVX vector carrying enhanced green fluorescent protein (eGFP) (negative control) or CSEPs, respectively, followed by inoculating with A. tumefaciens cells carrying PVX:BAX after 24 h. Photos were taken 5 days after infiltration.
HR Can Be Specifically Induced by CSEPs of P. triticina on Wheat Near-Isogenic Lines
According to the results of BAX assays, six CSEPs were chosen to verify the function using the transient expression infiltrated by A. tumefaciens method on wheat near-isogenic lines. The results showed that eGFP control cannot induce HR in any wheat near-isogenic lines, but chlorotic phenomenon was observed on wheat near-isogenic line leaves after infiltrated with CSEPs, including Pt7277, Pt88286, Pt3372 in TcLr42; Pt77192 in TcLr26; Pt36853 in TcLr47; and Pt16552 in TcLr41 (Figure 5). The results suggested that the overexpression of CSEPs of P. triticina can effectively induce HR.
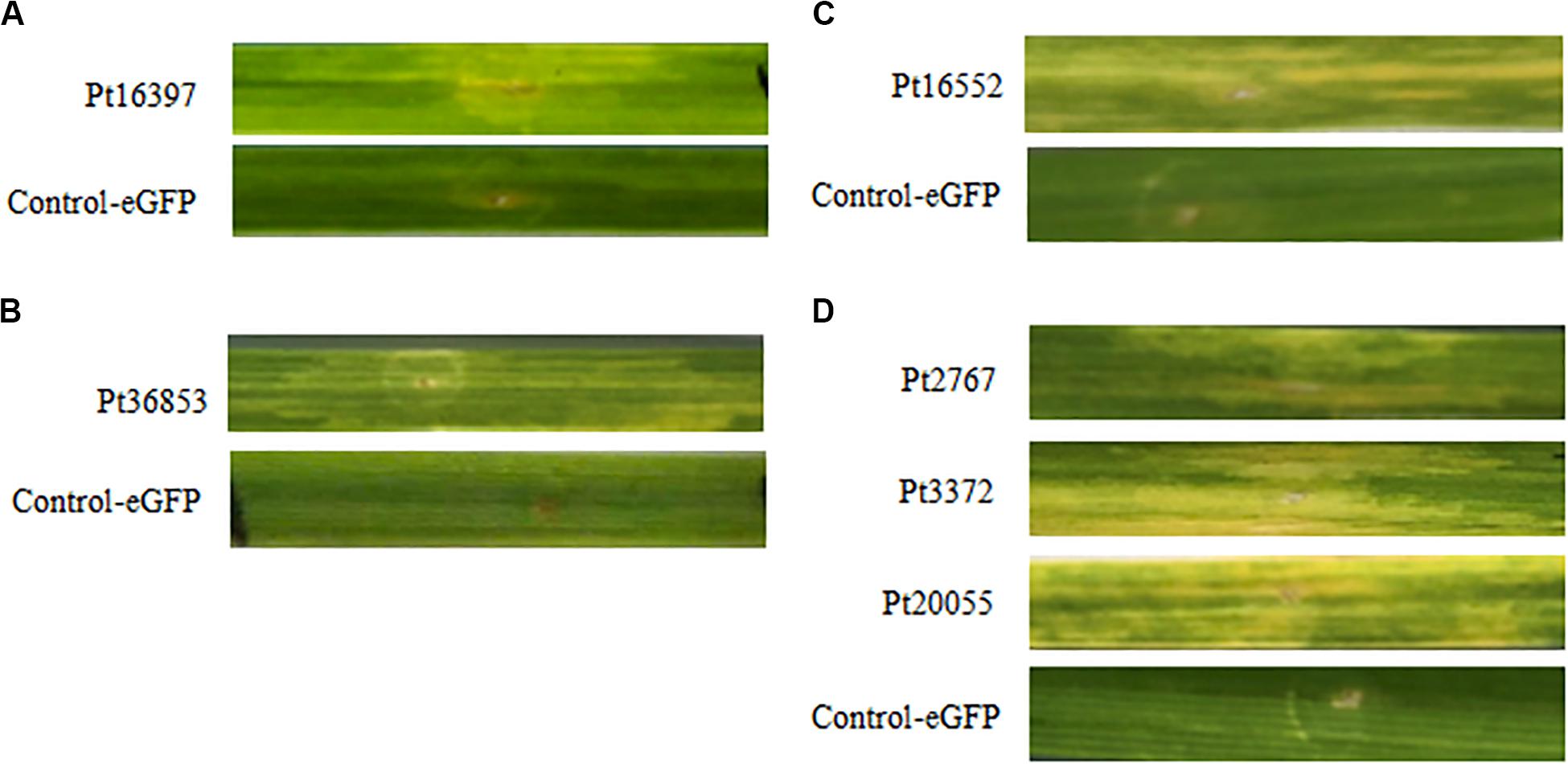
Figure 5. Six candidate secreted effector proteins (CSEPs) of P. triticina can especially induce hypersensitive response (HR) on the wheat near-isogenic lines. (A–C) Phenotype of Pt16397, Pt36853, and Pt16552 inoculated on wheat near-isogenic lines TcLr26, TcLr47, and TcLr41, respectively. (D) Pt7277, Pt88286, and Pt3372 play role in TcLr42.
The Expression Pattern of CSEPs of P. triticina at Different Development Stages
The expression characteristics of 14 CSEPs on “Thatcher” were analyzed by qRT-PCR. We found that Pt77192 was upregulated during 0–6 hpi and reached the peak at 6 hpi; then, it showed a significant downward trend when interacted with “Thatcher.” Pt5974 peaked at 12 hpi, and the expression was downregulated during 18–120 hpi. Pt34354 showed the highest expression at 18 hpi, then decreased, and increased at 36 and 60 hpi, respectively. Both Pt23713 and Pt1625 reached the expression peak at 36 hpi. Pt1625 decreased rapidly at 48 hpi after upregulating at 36 hpi and reached an expression peak at 60 hpi, followed by downregulation. Pt36553 was not expressed during 0–6 hpi, but it was expressed in wavy trend during 12–36 hpi, peaked at 48 hpi, then was downregulated at 96 hpi. Pt12858 was upregulated at 12 and 36 hpi and peaked at 96 hpi. Pt96482 showed almost no expression during 0–36 hpi but began to climb two peaks at 60 and 96 hpi, respectively. Pt18222, Pt29088, and Pt36853 reached the peak expression at 120 hpi. Pt18222 showed a low expression level at 0–96 hpi and peaked at 120 hpi. Pt29088 showed a low expression level during 0–60 hpi and gradually upregulated at 72 hpi until reaching the expression peak at 120 hpi. Pt36853 started to express at 72 hpi, and the highest expression level was at 120 hpi. The expressions of Pt20779, Pt16481, and Pt15525 showed the same trend. They all showed a wavy trend during 0–72 hpi, upregulated at 72 hpi, and then peaked at 120 hpi (Figure 6).
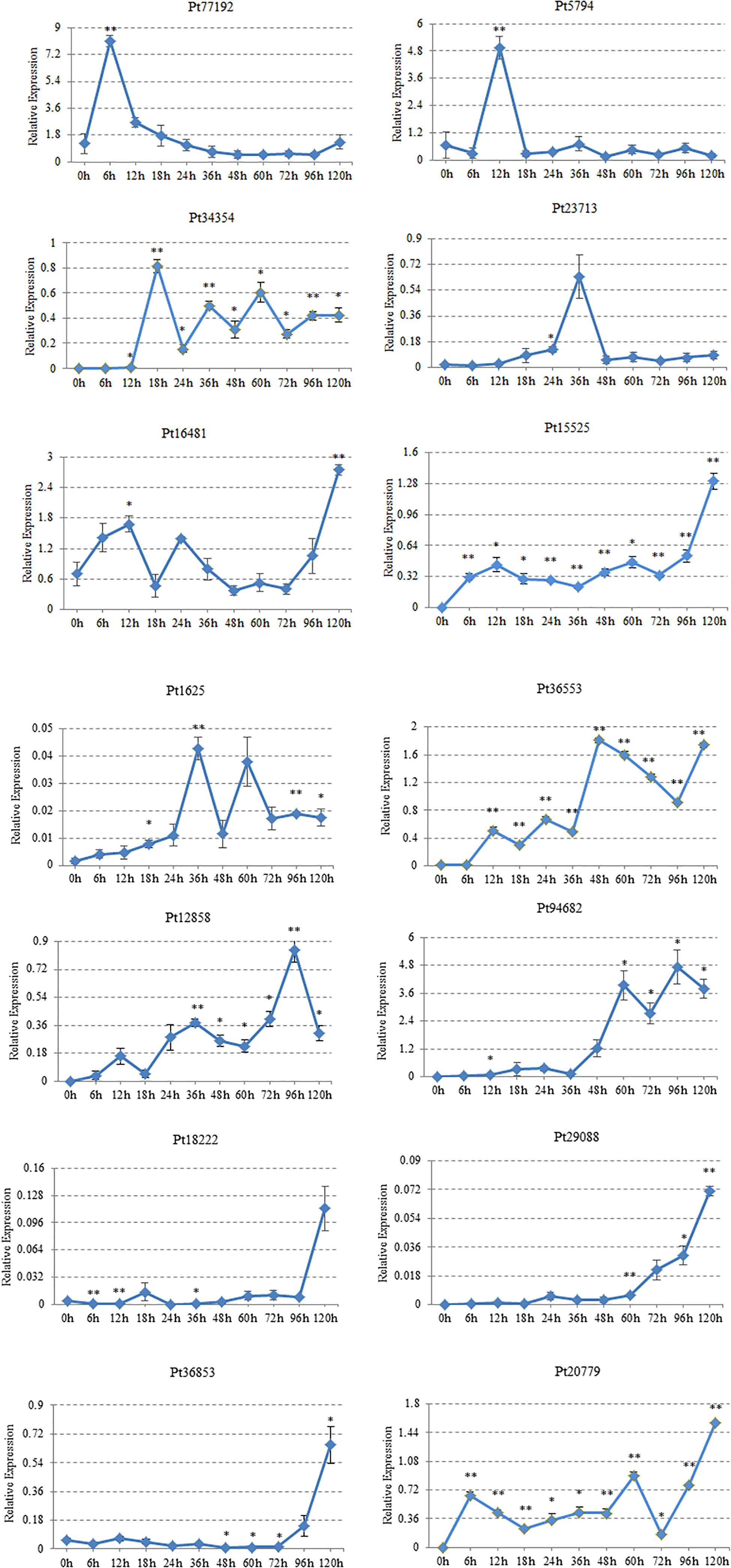
Figure 6. Gene expression profiles for 14 candidate effector proteins. Plant samples were taken at these inoculation time points (X-axis). h, hours post-inoculation (hpi). Gene expression level, relative expression (Y-axis). The expression levels of each gene were calculated according to the 2–ΔΔCT method compared with an endogenous gene EF1. Three technical replicates and biological repetition for each treatment were analyzed. Calculations of the mean and standard error were performed using Microsoft Excel software. Asterisks indicate significant differences (∗∗P < 0.01, ∗P < 0.05) relative to the US sample determined using Student’s t-test.
Discussion
Utilizing Multiple Approaches Can Improve the Prediction Efficiency and Accuracy of CSEPs
Effector proteins are important virulence or avirulence factors in pathogens. Some fungal CSEPs have certain characteristics, such as containing conservative motifs RxLR in oomycetes and [Y/F/W]xC in powdery mildew or have sequence similarity. However, most fungal effectors generally do not exhibit a high degree of amino acid sequence conservation. Therefore, the prediction of effector mainly depends on the method of bioinformatics. Previous study has verified that this method is useful to predict the secreted proteins of various pathogens (Sonah et al., 2016). The first step of the prediction of CSEPs is usually to predict extracellular secretion signals. SignalP v4.1 based on SP site combines with several artificial neural networks for predicting SP/non-SP. D-score values can be set (to distinguish between SP and non-SP values) as well as the minimum position in the sequence. The use of SignalP v4.1 makes the screening conditions more stringent and more accurate and greatly narrows the scope of secreted proteins.
Some CSEPs have plant localization signals; different effectors may localize to plant cell membranes, cytoplasm, endoplasmic reticulum, Golgi, mitochondria, chloroplast, and nucleus. TargetP can predict protein localization in large quantities, which provides valuable clues for determining the site of action of plant intracellular effector. The accuracy rate for plants and non-plants are up to 85 and 90%, respectively (Sonnhammer et al., 1998; Emanuelsson et al., 2000).
The presence of signal peptides and plant localization signals is not sufficient to indicate that a protein is secreted. SP can also direct proteins to cytoplasmic organelles. Therefore, distinguishing SP and TM is very important (Robinson et al., 2012; Reithinger et al., 2013). There are several predictive servers available for predicting TM regions. TMHMM is a tool for predicting transmembrane domains of proteins using hidden Markov Models. It covers the prediction of structural and chemical properties in the transmembrane region as well as inside and outside the membrane and predicts the charge, hydrophobicity, membrane protein topology, and helical length of the transmembrane region. The software can effectively distinguish SP and TM to improve prediction accuracy.
A number of effector proteins can be predicted using the above three software. A total of 4,514 candidate effectors were predicted based on SignalP v4.1, TargetP v1.1, and TMHMM v2.0. Based on the properties of the effector proteins, we further predicted them using EffectorP. EffectorP v1.0, an effector protein prediction software, was developed based on a series of properties of proteins. It is the first prediction program based on machine training to predict fungal effectors (Sperschneider et al., 2016). This program promotes functional studies of fungal effector and enhances our understanding of plant–pathogen interactions. However, EffectorP v1.0 uses a small range of screening criteria, including amino acid frequency, molecular weight, sequence length, and net protein charge. The software was optimized with adding filters based on the hydrophilicity, hydrophobicity, and surface tension of the proteins and developed into EffectorP v2.0 in 2018 (Sperschneider et al., 2018a). For the accuracy of the prediction of two versions, corresponding experiments were conducted. CSEPs of 13 fungi, such as Colletotrichum higginsianum, Cladosporium fulvum, M. oryzae, Blumeria graminis f. sp. hordei, Melampsora larici-populina, P. gramminis f. sp. tritici, Ustilago maydis, and Leptosphaeria maculans in the infection stage, were predicted (Sperschneider et al., 2018a). They found that 12 species were predicted by EffectorP v2.0, and the prediction rate reached to 92.3%. However, 10 species were predicted by EffectorP v1.0, and the forecast rate is 76.9%. At the same time, researchers combined the two versions to predict 115 effectors and found that the accuracy is as high as 90.5%, which is higher than the probability of using only EffectorP v1.0 or v2.0. The combination of the two versions is the most powerful means to predict effector, but numerous effector proteins are lost. Thus, the ability of EffectorP v2.0 to predict fungal effector proteins is higher than that of EffectorP v1.0, and the range of prediction is also beyond the combined two versions. Therefore, we chose EffectorP v2.0 for the effector protein prediction on the base of screening by SignalP v4.1, TargetP v1.1, and TMHMM v2.0. Finally, a total of 635 CSEPs were obtained. EffectorP v2.0 is a powerful tool for predicting fungal candidate secreted effector proteins, but more experiments are needed to support the prediction results, such as host-induced gene silencing (HIGS) and transgenosis, to discover the function of the effector. Moreover, yeast two-hybrid system (Y2H), bimolecular fluorescence complementation (BiFC), and immunoprecipitation are essential to identify the interaction target with host.
CSEPs of P. triticina Contained Some Known Motifs of Other Pathogens
Previous studies has confirmed that the effector proteins contained the motifs, such as RxLR in oomycetes, [L/I]xAR and YxSL[R/K] in rice blast fungus, [Y/F/W]xC in powdery mildew, and G[I/F/Y][A/L/S/T]R in flax rust. The consensus sequence RxLR motif was originally identified by comparing effectors from Hyaloperonospora arabidopsidis, Phytophthora infestans, and Phytophthora sojae but found no RxLR motif in fungal effectors (Rehmany et al., 2005). Interestingly, in this study, 24 CSEPs with RxLR motif were found from 635 CSEPs of P. triticina. Moreover, we found 244 candidate effector proteins with [Y/F/W]xC motif in powdery mildew, which is larger than 57 found in wheat leaf rust fungi by Godfrey et al. (2010). The results demonstrated that the effector proteins produced by P. triticina have similar structure as the B. graminis f. sp. hordei effectors. The CSEPs with [Y/F/W]xC motif accounted for 38.43% of all the CSEPs in our library, suggesting that the motif plays fundamental roles in haustoria function. Furthermore, five effector proteins contain G[I/F/Y][A/L/S/T]R motif, which was reported in flax rust. Two YxSL[R/K] motifs and 64 [L/I]xAR motifs in M. oryzae were found in P. triticina (Figure 1). No known motifs were found in the other 296 CSEPs, representing 46.6% of all the CSEPs (Figure 1), indicating that the CSEPs of P. triticina have certain sequence and hereditary homology with the known fungal effector proteins. This also indicated that the CSEPs of P. triticina predicted by EffectorP v2.0 were accurate.
CSEPs of P. triticina Contained New Motifs and Homology to P. gramminis f. sp. tritici and P. striiformis f. sp. tritici
Three kinds of motifs that differed from other fungi were first discovered by MEME prediction for 296 CSEPs that have no known motifs (Figure 3), indicating that the CSEPs in P. triticina are diverse and specific. However, the function of the known motif remains to be confirmed experimentally.
To demonstrate the accuracy of the results, we also blast the 635 CSEPs with secreted proteins of P. triticina, P. gramminis f. sp. tritici, and P. striiformis f. sp. tritici reported by Cantu et al. (2013) using TBtools v0.66839 (Chen et al., 2018). The results showed that 339 genes were identical to genes in BBBD isolate of P. triticina. In addition, 90 and 54 candidate effector proteins were identical to sequences in P. gramminis f. sp. tritici and P. striiformis f. sp. tritici, respectively, and the identities ranged from 70 to 90%. The results indicated that the CSEPs of P. triticina were related to that in other rust fungi, and they were more specific and difficult to obtain by homologous sequence method.
Structural features of fungal effector proteins include extracellular localization, small sequence, or low molecular weight (e.g., sequences are < 300 AA or sequences are <30 kDa) and enriched in cysteine (e.g., sequences contain more than or equal to four cysteine). Figueroa et al. (2015) found that effector ToxB had 87 amino acids and 4 cysteines in Pyrenophora tritici-repentis. However, previous studies found that there are some exceptions in the CSEPs. For example, the P. sojae effector protein PsXEG1 contains only two cysteines (Ma et al., 2015). The flax rust effector protein AvrM has 314 amino acids but only 1 cysteine (Catanzariti et al., 2006). In this study, we predicted 635 CSEPs, and most of them were conventional. The number of cysteine residues in 427 genes were ≥4, especially Pt44738 that contained 28 cysteines. The remaining 208 genes contained less than four cysteines. The molecular weight of 625 out of 635 candidate effector proteins were < 300 amino acids. However, the size of 10 genes were >300 amino acids. For example, the molecular weight of Pt91311 were 422 amino acids, indicating that the CSEPs of P. triticina has abundant specificity. Therefore, screening in a broad condition may filter out some effectors. More experimental data are needed to optimize the screening method, such as using the known effector information, expanding screening criteria, etc.
CSEPs of P. triticina May Confer Many Functions
At present, some CSEPs in the plant phytopathology have classified biological functions. Based on the Pfam domain search, we found some related families and domains in this study. Among them, 27 genes were found to belong to the hydrolase family, including the glycosyl hydrolase family and the dienelactone hydrolase family. There were 10 CSEPs assigned to the known proteins with hydrolase (Cuomo et al., 2017). Previous studies suggested that hydrolases such as glucanase and chitinase were involved in the fungal spore germination process (Mouyna et al., 2013), hypha growth and branching emergence (Wessels, 1993; Adams et al., 2004; Sandini et al., 2007), hyphae fusion (Glass et al., 2000), basidiomycete fruiting development (Kamada and Takemaru, 1977; Sakamoto et al., 2005; Tao et al., 2013), and the autolysis acting in releasing the fruiting bodies of the spores (Kües, 2000; Liu et al., 2015). These are all related to the growth and development of fungi.
Another large group found in six CSEPs belonged to the Thaumatin family. Grenier et al. (2000) first reported this family in basidiomycetes. The gene encoded by the Thaumatin-like protein (TLP) was found in Cryptococcus neoformans and participated in the degradation of the plant lentinan and the cell wall during the microspore diffusion process (Sakamoto et al., 2006). Among the 1,358 wheat leaf rust CSEPs (Cuomo et al., 2017), six genes contain thaumatin domain, but their functions have not been reported. In our research, 11 genes belonged to the Barwin family (Table 1) known elicitor cerato-platanin of fungi (Pazzagli et al., 1999), which are more than that found by Bruce et al. (2014), who found only one CSEP that was associated with Barwin through performing transcriptome analysis on 6 P. triticina races and obtained 532 CSEPs. It has also been reported that proteins containing this domain promoted the successful colonization of C. neoformans in the lungs of mammals (Camacho et al., 2019). In addition, these 635 candidate effector proteins included other family domains (Table 1), such as the ribonuclease family, the peptidase family, the aldehyde dehydrogenase family, the Bowman–Birk serine protease inhibitor family, the Ras family, etc. However, the functions of these families in fungi need to be further explored.
We also found 9 domains in the 635 CSEPs (Table 1), of which the CFEM domain is an extracellular plasma membrane protein containing eight cysteine residues, a unique protein associated with fungal pathogenesis. There are other enzymes and proteins (Supplementary Figure S2), such as copper/zinc superoxide dismutase, polysaccharide deacetylase, hydrophobic protein, phytosulfokine precursor protein, etc. They may play a role in the interaction between pathogens and hosts, but the functions of these conserved domains need to be further studied.
Heterologous Transient Expression Verified the CSEPs of P. triticina
P. triticina is an obligate biotrophic fungus, and it lacks an effective genetic transformation system to be verified in vitro. Therefore, Agrobacterium-mediated transient transformation system was applied in assessing the function of effector. Researches had shown that cell necrosis induced by BAX in plants was similar to the HR induced by pathogen in physiological characteristics (Lacomme and Santa Cruz, 1999). It is speculated that the inhibition of BAX-induced PCD reflected the virulent function of inhibiting host defense response of CSEPs. It is a simple and effective way to identify the function of effector. Our experiment utilized heterologous expression system and the mouse-apoptotic gene BAX elicitor to detect the function of the 30 CSEPs of P. triticina in N. benthamiana 5 days after inoculation with A. tumefaciens. The results showed that 30 CSEPs of P. triticina that we tested inhibited BAX-induced PCD, and the inhibition ratio was up to 100%; this hints that the screened CSEPs of P. triticina are more accurate.
Instantaneous Expression of the CSEPs on Wheat Near-Isogenic Lines
We conducted cell-death suppression assay for selected 30 CSEPs using BAX elicitor in vitro. However, these results are still indirect evidence. Therefore, we used A. tumefaciens-mediated instantaneous expression to directly test wheat near-isogenic lines. The results showed that six CSEPs of P. triticina can especially induce HR on different wheat cultivars. Pt77192, Pt36853, and Pt16552 acted in TcLr26, TcLr47, and TcLr41, respectively. Interestingly, Pt7277, Pt88286, and Pt3372 played role in TcLr42 at the same time. The reason why different effectors from the same P. triticina could stimulate the same R protein is that effector redundancy is a product of arms race coevolution between pathogens and hosts; they may be recognized by the R protein Lr42 in different ways, such as the guard mode or decoy mode. In biology, genetic redundancy contributes to robustness in the face of a changing environment. Similarly, plant–pathogen populations benefit from carrying a redundant reservoir of effectors to counteract host immunity (Win et al., 2012). This could also explain why different effectors can target the same host protein whether the effectors are related.
qRT-PCR Showed the Expression Characteristics of CSEPs of Wheat Leaf Rust
The production and expression of CSEPs differed at different stages. Real-time quantitative expression analysis can reveal the expression characteristics of CSEPs, which help to prove that CSEPs are effectors indirectly through the expression time points. In this study, there were 14 genes in the stage of P. triticina infecting on wheat analyzed by qRT-PCR. According to the results of transcriptome sequencing, there were 14 genes upregulated in the germination stage and the infection stage of P. triticina race JHKT. From the results of qRT-PCR, the expression levels of Pt18222, Pt29088, and Pt36853 reached the peak at 120 hpi, while Pt12858 and Pt96482 showed the highest expression at 96 hpi. Pt20779, Pt16481, and Pt15525 were expressed in the whole stage of interaction between leaf rust and host. Pt77192, Pt5974, Pt34354, Pt23713, Pt1625, and Pt36553 were expressed at 6, 12, 18, 36, and 48 hpi. These results indicated that these genes were induced when interacting with the host and were upregulated in the different interaction stages. Therefore, they may act as candidate effector proteins and may play different roles in the pathogenic process.
The Future of Prediction of Fungal Effector Proteins
In this research, we chose the CDS in the cDNA library obtained by RNA-seq sequencing to screen the effectors; this may not be a full-length sequence due to splicing, de-redundancy, etc. Therefore, the results predicted by software alone are not all of the CSEPs of pathogens. It is necessary to fully understand CSEPs with a large number of related studies of different pathogenic strains. Although we use the multiple prediction approaches to predict the effectors in P. triticina during the different interaction stages with wheat cultivar “Thatcher,” structural analysis, heterologous expression, and other methods are used to improve the efficiency and accuracy of the CSEPs screening of wheat leaf rust. Some CSEPs were lost due to the existence of specificity of candidate secreted effector proteins. For example, researchers found that some effector proteins have no signal peptide (Prasad et al., 2019). Additionally, the tools that we used were based on the characters of the cloned effector proteins, so further functional verification is required. It is believed that along with the deepening of research and the improvement of methods, the CSEPs will be enriched and improved.
Conclusion
Integrated software including SignalP v4.1, TargetP v1.1, TMHMM v2.0, and EffectorP v2.0 for analyzing the RNA-seq data are efficient in predicting CSEPs, and 635 CSEPs were screened from RNA-seq data of 3 P. triticina strains – KHTT, JHKT, and THSN – at three different stages in this way. The CSEPs of P. triticina contain various family domains including glycosyl hydrolase family, Thaumatin family, Barwin family, etc. Several known motifs such as RxLR, [Y/F/W]xC, G[I/F/Y] [A/L/S/T]R, [L/I]xAR, and YxSL[R/K] and three de novo motifs were identified. CSEPs found in P. triticina have above 70% identity to the sequences in P. gramminis f. sp. tritici and P. striiformis f. sp. tritici. The tested CESPs can inhibited BAX-induced PCD and the inhibition ratio up to 100%. The results laid a solid foundation for understanding the interaction mechanism between P. triticina and wheat, enriching the pathogenic mechanism of obligate biotrophic fungal and utilizing avirulent genes.
Data Availability Statement
The datasets presented in this study can be found in online repositories. The data has deposited in the NCBI. The SRA accession numbers are SRR11929934, SRR11929933, SRR11929922, SRR11929915, SRR11929914, SRR11929913, SRR11929912, SRR11929911, SRR11929910, SRR11929909, SRR11929932, SRR11929931, SRR11929930, SRR11929929, SRR11929928, SRR11929927, SRR11929926, SRR11929925, SRR11929924, SRR11929923, SRR11929921, SRR11929920, SRR11929919, SRR11929918, SRR11929917, and SRR11929916. The TSA accession number is GISY00000000 and consists of sequences and consists of sequences GISY01000001–GISY01092094.
Author Contributions
YZ, WY, and DL contributed to the design of the work. WY supervised the experiments. JW constructed RNA-Seq library. YZ analyzed the sequencing data. YZ and NZ submitted the data to NCBI. YZ, YQ, and JL performed the experiments. YZ wrote the manuscript. JW, RA, NZ, and WY revised the manuscript.
Funding
This work was funded by the National Natural Science Foundation of China (Grant Nos. 301571956 and 301871915), National Key R&D Research Program of China (Grant No. 2017YFD70700), and Modern Agricultural Industry System of Wheat Industry in Hebei Province (Grant No. HBCT2018010204).
Conflict of Interest
The authors declare that the research was conducted in the absence of any commercial or financial relationships that could be construed as a potential conflict of interest.
Acknowledgments
We would like to thank Prof. Sun Wenxian of China Agricultural University for providing the GV3101 isolates for this work. Thanks to Prof. Shen Qianhua from the Institute of Genetics and Developmental Biology, Chinese Academy of Sciences for providing N. benthamiana seeds.
Supplementary Material
The Supplementary Material for this article can be found online at: https://www.frontiersin.org/articles/10.3389/fmicb.2020.538032/full#supplementary-material
FIGURE S1 | The flow chart of the prediction of candidate secreted effector proteins.
FIGURE S2 | Domains of other known functions of 635 candidate effector proteins.
FIGURE S3 | Thirty candidate effector proteins that can inhibit the cell death triggered by BAX. 1–30 represents the candidate effector proteins Pt7277, Pt10853, Pt12858, Pt16481, Pt5794, Pt494, Pt3081, Pt97002, Pt15387, Pt34354, Pt88286, Pt15525, Pt8502, Pt15546, Pt20779, Pt8638, Pt77192, Pt1625, Pt23713, Pt36553, Pt18222, Pt36853, Pt2567, Pt94682, Pt29088, Pt16552, Pt16, Pt17, Pt3372, Pt14306.
TABLE S1 | Primers used in the HR suppression assays.
TABLE S2 | Primers used in the qRT-PCR assays.
Footnotes
- ^ http://www.cbs.dtu.dk/services/SignalP/
- ^ http://www.cbs.dtu.dk/services/TargetP/
- ^ http://www.cbs.dtu.dk/services/TMHMM/
- ^ http://effectorp.csiro.au/
- ^ https://dataview.ncbi.nlm.nih.gov/object/PRJNA609405?reviewer=j2png1bo7u3jlpp6h3amhk0jfm
References
Adams, D. J. (2004). Fungal cell wall chitinases and glucanases. Microbiology 150, 2029–2035. doi: 10.1099/mic.0.26980-0
Bendtsen, J. D., Nielsen, H., Von Heijne, G., and Brunak, S. (2004). Improved prediction of signal peptides: signalP 3.0. J. Mol. Biol. 340, 783–795. doi: 10.1016/j.jmb.2004.05.028
Bruce, M., Neugebauer, K. A., Joly, D. L., Migeon, P., Cuomo, C. A., Wang, S., et al. (2014). Using transcription of six Puccinia triticina races to identify the effective secretome during infection of wheat. Front. Plant Sci. 4:520. doi: 10.3389/fpls.2013.00520
Camacho, E., Vij, R., Chrissian, C., Pradosrosales, R., Gil, D., O’meally, R. N., et al. (2019). The structural unit of melanin in the cell wall of the fungal pathogen Cryptococcus neoformans. J. Biol. Chem. 294, 10471–10489. doi: 10.1074/jbc.RA119.008684
Cantu, D., Segovia, V., Maclean, D., Bayles, R., Chen, X., Kamoun, S., et al. (2013). Genome analyses of the wheat yellow (stripe) rust pathogen Puccinia striiformis f. sp. tritici reveal polymorphic and haustorial expressed secreted proteins as candidate effectors. BMC Genom. 14:270. doi: 10.1186/1471-2164-14-270
Catanzariti, A. M., Dodds, P. N., Lawrence, G. J., Ayliffe, M. A., and Ellis, J. G. (2006). Haustorially expressed secreted proteins from flax rust are highly enriched for avirulence elicitors. Plant Cell 18, 243–256. doi: 10.1105/tpc.105.035980
Chen, C., Chen, H., He, Y., and Xia, R. (2018). TBtools, a toolkit for biologists integrating various HTS-data handling tools with a user-friendly interface. bioRxiv [Preprint], doi: 10.1101/289660
Chen, J., Upadhyaya, N. M., Ortiz, D., Sperschneider, J., Li, F., Bouton, C., et al. (2017). Loss of AvrSr50 by somatic exchange in stem rust leads to virulence for Sr50 resistance in wheat. Science 358, 1607–1610. doi: 10.1126/science.aao4810
Cuomo, C. A., Bakkeren, G., Khalil, H. B., Panwar, V., Joly, D., Linning, R., et al. (2017). Comparative analysis highlights variable genome content of wheat rusts and divergence of the mating loci. G3 Genes Genet. 7, 361–376. doi: 10.1534/g3.116.032797
Dalio, R. J., Herlihy, J., Oliveira, T. S., Mcdowell, J. M., and Machado, M. (2017). Effector biology in focus: a primer for computational prediction and functional characterization. Mol. Plant Microb. Interact. 31, 22–33. doi: 10.1094/MPMI-07-17-0174-FI
Dodds, P. N., Lawrence, G. J., Catanzariti, A. M., Ayliffe, M. A., and Ellis, J. G. (2004). The Melampsora lini AvrL567 avirulence genes are expressed in haustoria and their products are recognized inside plant cells. Plant Cell 16, 755–768. doi: 10.1105/tpc.020040
D’Silva, I., and Heath, M. C. (1997). Purification and characterization of two novel hypersensitive response-inducing specific elicitors produced by the cowpea rust fungus. J. Biol. Chem. 272, 3924–3927. doi: 10.1074/jbc.272.7.3924
Emanuelsson, O., Nielsen, H., Brunak, S., and Von Heijne, G. (2000). Predicting subcellular localization of proteins based on their N-terminal amino acid sequence. J. Mol. Biol. 300, 1005–1016. doi: 10.1006/jmbi.2000.3903
Figueroa, M., Manning, V. A., Pandelova, I., and Ciuffetti, L. M. (2015). Persistence of the host-selective toxin Ptr ToxB in the apoplast. Mol. Plant Microb. Interact. 28, 1082–1090. doi: 10.1094/MPMI-05-15-0097-R
Glass, N. L., Jacobson, D. J., and Shiu, P. K. (2000). The genetics of hyphal fusion and vegetative incompatibility in filamentous ascomycete fungi. Annu. Rev. Phytopathol. 34, 165–186. doi: 10.1146/annurev.genet.34.1.165
Godfrey, D., Böhlenius, H., Pedersen, C., Zhang, Z., Emmersen, J., and Thordal-Christensen, H. (2010). Powdery mildew fungal effector candidates share N-terminal Y/F/WxC-motif. BMC Genom. 11:317. doi: 10.1186/1471-2164-11-317
Grenier, J., Potvin, C., and Asselin, A. (2000). Some fungi express β-1, 3-glucanases similar to thaumatin-like proteins. Mycologia 92, 841–848. doi: 10.2307/3761579
Han, D. J., Cao, L., Chen, Y. F., and Li, Z. Q. (2005). Molecular basic of interaction between disease resistance gene and avirulence gene. Acta Genet. Sin. 32, 1319–1326.
Kamada, T., and Takemaru, T. (1977). Stipe elongation during basidiocarp maturation in Coprinus macrorhizus: mechanical properties of stipe cell wall. Plant Cell Physiol. 18, 831–840. doi: 10.1093/oxfordjournals.pcp.a075498
Kamoun, S. (2006). A catalogue of the effector secretome of plant pathogenic oomycetes. Annu. Rev. Phytopathol. 44, 41–60. doi: 10.1146/annurev.phyto.44.070505.143436
Krogh, A., Larsson, B., Von Heijne, G., and Sonnhammer, E. L. (2001). Predicting transmembrane protein topology with a hidden Markov model: application to complete genomes. J. Mol. Biol. 305, 567–580. doi: 10.1006/jmbi.2000.4315
Kües, U. (2000). Life history and developmental processes in the basidiomycete Coprinus cinereus. Microbiol. Mol. Biol. Rev. 64, 316–353. doi: 10.1128/MMBR.64.2.316-353.2000
Lacomme, C., and Santa Cruz, S. (1999). Bax-induced cell death in tobacco is similar to the hypersensitive response. Proc. Natl. Acad. Sci. U.S.A. 96, 7956–7961. doi: 10.1073/pnas.96.14.7956
Liu, Z., Niu, X., Wang, J., Zhang, W., Yang, M., Liu, C., et al. (2015). Comparative study of nonautolytic mutant and wild-type strains of Coprinopsis cinerea supports an important role of glucanases in fruiting body autolysis. J. Agric. Food Chem. 63, 9609–9614. doi: 10.1021/acs.jafc.5b03962
Livak, K. J., and Schmittgen, T. D. (2001). Analysis of relative gene expression data using real-time quantitative PCR and the 2−ΔΔCT method. Methods 25, 402–408. doi: 10.1006/meth.2001.1262
Lo Presti, L., Lanver, D., Schweizer, G., Tanaka, S., Liang, L., Tollot, M., et al. (2015). Fungal effectors and plant susceptibility. Annu. Rev. Phytopathol. 66, 513–545. doi: 10.1146/annurev-arplant-043014-114623
Ma, Z., Song, T., Zhu, L., Ye, W., and Wang, Y. (2015). A Phytophthora sojae glycoside hydrolase 12 protein is a major virulence factor during soybean infection and is recognized as a PAMP. Plant Cell 27, 2057–2072. doi: 10.1105/tpc.15.00390
Möller, S., Croning, M. D., and Apweiler, R. (2001). Evaluation of methods for the prediction of membrane spanning regions. Bioinformatics 17, 646–653. doi: 10.1093/bioinformatics/17.7.646
Mouyna, I., Hartl, L., and Latgé, J. P. (2013). β-1,3-glucan modifying enzymes in Aspergillus fumigatus. Front. Microbiol. 4:81. doi: 10.3389/fmicb.2013.00081
Nielsen, H. B. (2017). Predicting secretory proteins with SignalP. Methods Mol. Biol. 1611, 59–73. doi: 10.1007/978-1-4939-7015-5_6
Pazzagli, L., Cappugi, G., Manao, G., Camici, G., Santini, A., and Scala, A. (1999). Purification, characterization, and amino acid sequence of cerato-platanin, a new phytotoxic protein from Ceratocystis fimbriata f. sp. platani. J. Biol. Chem. 274, 24959–24964. doi: 10.1074/jbc.274.35.24959
Prasad, P., Savadi, S., Bhardwaj, S., Gangwar, O., and Kumar, S. (2019). Rust pathogen effectors: perspectives in resistance breeding. Planta 250, 1–22. doi: 10.1007/s00425-019-03167-6
Rehmany, A. P., Gordon, A., Rose, L. E., Allen, R. L., Armstrong, M. R., Whisson, S. C., et al. (2005). Differential recognition of highly divergent downy mildew avirulence gene alleles by RPP1 resistance genes from two Arabidopsis lines. Plant Cell 17, 1839–1850. doi: 10.1105/tpc.105.031807
Reithinger, J. H., Kim, J. E. H., and Kim, H. (2013). Sec62 protein mediates membrane insertion and orientation of moderately hydrophobic signal anchor proteins in the endoplasmic reticulum (ER). J. Biol. Chem. 288, 18058–18067. doi: 10.1074/jbc.M113.473009
Robinson, P. J., Findlay, J. E., and Woolhead, C. A. (2012). Compaction of a prokaryotic signal-anchor transmembrane domain begins within the ribosome tunnel and is stabilized by SRP during targeting. J. Mol. Biol. 423, 600–612. doi: 10.1016/j.jmb.2012.07.023
Sakamoto, Y., Irie, T., and Sato, T. (2005). Isolation and characterization of a fruiting body-specific exo-β-1,3-glucanase-encoding gene, exg1, from Lentinula edodes. Curr. Genet. 47, 244–252. doi: 10.1007/s00294-005-0563-7
Sakamoto, Y., Watanabe, H., Nagai, M., Nakade, K., Takahashi, M., and Sato, T. (2006). Lentinula edodes tlg1 encodes a thaumatin-like protein that is involved in lentinan degradation and fruiting body senescence. Plant Physiol. 141, 793–801. doi: 10.1104/pp.106.076679
Salcedo, A., Rutter, W., Wang, S., Akhunova, A., Bolus, S., Chao, S., et al. (2017). Variation in the AvrSr35 gene determines Sr35 resistance against wheat stem rust race Ug99. Science 358, 1604–1606. doi: 10.1126/science.aao7294
Sandini, S., La Valle, R., De Bernardis, F., Macrì, C., and Cassone, A. (2007). The 65 kDa mannoprotein gene of Candida albicans encodes a putative β-glucanase adhesin required for hyphal morphogenesis and experimental pathogenicity. Cell Microbiol. 9, 1223–1238. doi: 10.1111/j.1462-5822.2006.00862.x
Shan, W., Cao, M., Leung, D., and Tyler, B. M. (2004). The Avr1b locus of Phytophthora sojae encodes an elicitor and a regulator required for avirulence on soybean plants carrying resistance gene Rps 1b. Mol. Plant Microb. Interact. 17, 394–403. doi: 10.1094/MPMI.2004.17.4.394
Sonah, H., Deshmukh, R. K., and Bélanger, R. R. (2016). Computational prediction of effector proteins in fungi: opportunities and challenges. Front. Plant Sci. 7:126. doi: 10.3389/fpls.2016.00126
Sonnhammer, E. L., Von Heijne, G., and Krogh, A. (1998). A hidden markov model for predicting transmembrane helices in protein sequences. Proc. Int. Conf. Intell. Syst. Mol. Biol. 6, 175–182.
Sperschneider, J., Dodds, P. N., Gardiner, D. M., Manners, J. M., Singh, K. B., and Taylor, J. M. (2015). Advances and challenges in computational prediction of effectors from plant pathogenic fungi. PLoS Pathog. 11:e1004806. doi: 10.1371/journal.ppat.1004806
Sperschneider, J., Dodds, P. N., Gardiner, D. M., Singh, K. B., and Taylor, J. M. (2018a). Improved prediction of fungal effector proteins from secretomes with EffectorP 2.0. Mol. Plant Pathol. 19, 2094–2110. doi: 10.1111/mpp.12682
Sperschneider, J., Gardiner, D. M., Dodds, P. N., Tini, F., Covarelli, L., Singh, K. B., et al. (2016). EffectorP: predicting fungal effector proteins from secretomes using machine learning. New Phytol. 210, 743–761. doi: 10.1111/nph.13794
Sweigard, J. A., Carroll, A. M., Kang, S., Farrall, L., Chumley, F. G., and Valent, B. (1995). Identification, cloning, and characterization of PWL2, a gene for host species specificity in the rice blast fungus. Plant Cell 7, 1221–1233. doi: 10.1105/tpc.7.8.1221
Tao, Y., Xie, B., Yang, Z., Chen, Z., Chen, B., Deng, Y., et al. (2013). Identification and expression analysis of a new glycoside hydrolase family 55 exo-β-1,3-glucanase-encoding gene in Volvariella volvacea suggests a role in fruiting body development. Gene 527, 154–160. doi: 10.1016/j.gene.2013.05.071
Wessels, J. G. (1993). Fruiting in the higher fungi. Adv. Microb. Physiol. 34, 147–202. doi: 10.1016/S0065-2911(08)60029-6
Keywords: Puccinia triticina, effector proteins, BAX, qRT-PCR, RNA sequencing
Citation: Zhang Y, Wei J, Qi Y, Li J, Amin R, Yang W and Liu D (2020) Predicating the Effector Proteins Secreted by Puccinia triticina Through Transcriptomic Analysis and Multiple Prediction Approaches. Front. Microbiol. 11:538032. doi: 10.3389/fmicb.2020.538032
Received: 26 February 2020; Accepted: 26 August 2020;
Published: 22 September 2020.
Edited by:
Dilip Shah, Donald Danforth Plant Science Center, United StatesReviewed by:
Chuntao Yin, Washington State University, United StatesGeorgios Tzelepis, Swedish University of Agricultural Sciences, Sweden
Copyright © 2020 Zhang, Wei, Qi, Li, Amin, Yang and Liu. This is an open-access article distributed under the terms of the Creative Commons Attribution License (CC BY). The use, distribution or reproduction in other forums is permitted, provided the original author(s) and the copyright owner(s) are credited and that the original publication in this journal is cited, in accordance with accepted academic practice. No use, distribution or reproduction is permitted which does not comply with these terms.
*Correspondence: Wenxiang Yang, wenxiangyang2003@163.com; Daqun Liu, liudaqun@caas.cn
†These authors have contributed equally to this work